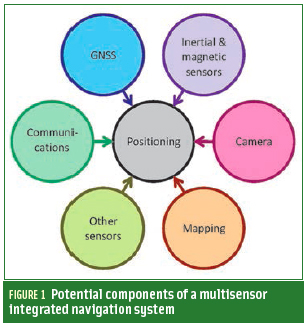
The navigation world is booming with new ideas at the moment to meet some of the greatest positioning challenges of our times. To realize demanding applications — such as reliable pedestrian navigation, lane identification, and robustness against interference, jamming and spoofing — we need to bring these different ideas together.
The navigation world is booming with new ideas at the moment to meet some of the greatest positioning challenges of our times. To realize demanding applications — such as reliable pedestrian navigation, lane identification, and robustness against interference, jamming and spoofing — we need to bring these different ideas together.
In this article, I want to explore 10 trends — some familiar, some new — that, taken as a whole, could have as much impact on our lives as the introduction of GNSS. They are cameras for navigation, cheaper and smaller sensors, multi-GNSS signals and systems, communications signals for positioning, 3-D mapping, multisensor navigation, context, opportunism, cooperation, and integrity.
Cameras, Cameras, Everywhere
The major new navigation sensor of the next decade could well be the camera. Although visual navigation systems have been around since the 1990s, their hardware cost and the processing power required to interpret the images limited their adoption.
Now, cheap digital cameras are ubiquitous. Cameras are standard equipment on mobile phones and autonomous vehicles and becoming commonplace in cars. In hardware terms, it is just a case of interfacing the camera(s) that are already there with the navigation system.
Moreover, over the past decade image processing and the computational capacity of mobile platforms have advanced dramatically, as has camera-based research within the navigation and positioning community.
A camera is a highly versatile sensor and can be used for navigation in at least three different ways.
The simplest method is to compare the camera’s image with a series of stored images in order to determine the camera’s viewpoint. Rather than comparing whole images, the camera extracts and compares a series of feature descriptors. This reduces processing and storage requirements substantially.
A more sophisticated position-fixing approach identifies individual features within an image. A camera is essentially a direction sensor; so, the camera determines position and orientation by measuring the directions to multiple features and intersecting lines of position through those features.
Both of these position-fixing techniques require a comparison of image features with stored information. The greater the initial position uncertainty, the longer it will take to perform the comparison and the greater the chance of a false or ambiguous match. Therefore, camera-based position fixing is best used as part of an integrated system in which GNSS or Wi-Fi or another technology provides an approximate position solution that is then refined by position date from the camera.
The third technique, visual odometry, is a form of dead reckoning. A camera’s motion can be inferred by comparing successive images. However, a camera senses angles, not distance; so, this technique needs additional scaling information to determine velocity from the image flow. Sometimes it can be difficult to distinguish linear from rotational motion.
Where there is space to mount a second camera, photogrammetry (stereo imagery) can resolve these problems. Otherwise, visual odometry must be combined with other navigation sensors to provide the necessary calibration.
Micro-Sensors, Radar and More
For ten years, research has promised to produce accelerometers, gyroscopes, and clocks that do the job at a lower cost, size, weight, and power consumption. These efforts will mature eventually.
Meanwhile, improved filtering and calibration and deploying sensor arrays should improve the performance of existing inertial sensors as well.
The U.S. Defense Advanced Research Projects Agency (DARPA) is investing in new micro-sensor technology to reduce military vulnerability to GPS jamming. Cold-atom interferometry is being tested for submarine navigation, and chip-scale atomic clocks are now available commercially.
Magnetometers won’t see much improvement because they are already available at low cost and their performance isn’t as critical to attitude determination accuracy as the much greater effects created by environmental magnetic anomalies and host vehicle magnetism.
Radar has been used for air and sea navigation for many decades and can provide both dead reckoning and position fixes. Radar systems used for collision avoidance in autonomous land vehicles, and now proposed for ordinary cars, could also be used for navigation.
3D laser imaging offers a higher resolution than radar, but the range is shorter. Scanning lasers provide the highest resolution, while flash LIDAR offers a faster update rate and lower cost, size, and power consumption.
GNSS and the Perils of Success
New GNSS satellite constellations, signals, and associated frequency diversity is stimulating innovations in user equipment design leading to improved capabilities for calibrating ionospheric propagation delays, robustness against incidental interference, and better accuracy from higher chipping rate signals.
Eventually, the quadrupling of available GNSS satellites will mean more signals to support autonomous integrity monitoring in open environments. In difficult environments, only those satellites with the best reception conditions need be used in the navigation solution.
User equipment will be able to exclude signals contaminated by multipath interference and non-line-of-sight reception if detected. Detection techniques include multi-frequency signal-to-noise comparisons, inter-satellite consistency checking, and the use of a dual-polarization antenna.
However, even with four satellite constellations, there will always be places — for example, in urban canyons or indoors — where it is impossible to receive direct line-of-sight signals from four satellites.
Unfortunately, success creates its own problems. Deliberate jamming, for example, is expected to become more prevalent. The motivation for using jammers, which are already widely available, will grow as such applications as GNSS-based road user charges and surveillance become more widespread.
Similarly, incidental interference can only get worse as the demand for spectrum increases. The GNSS community may have won the recent LightSquared battle, but the spectrum war is by no means over.
At the same time, GNSS modernization will improve the performance of many existing interference mitigation techniques. Combined signal and vector tracking and acquisition techniques work better with more signals and satellites. And extending the coherent integration interval to improve receiver sensitivity becomes quite a bit easier with the new data-free signals.
However, for maximum resistance to jamming and interference, we will always have to integrate GNSS with other positioning and navigation technologies.
Communications and the Positioning Algorithm
Arguably, the greatest innovation in navigation technology over the past decade was the use of com-munications signals for positioning purposes. Smartphones and other consumer devices now use phone signal and Wi-Fi positioning. Ultrawideband (UWB) communication signals are also used in specialist positioning applications, although customized UWB positioning systems perform better.
As new communications standards are introduced over the coming years, new positioning techniques will accompany them. The next wave of communications-based positioning systems will be based on fourth-generation phone signals, Bluetooth low energy, and Iridium satellite communications.
The first generation of communications-based positioning technologies had to cope with communications protocols that were not designed with positioning in mind. This typically limited the accuracy that could be achieved using standard equipment. Furthermore, the design of classic Bluetooth made it very difficult to use it for navigation at all.
Nowadays, new communications standards commonly incorporate ranging protocols. In future systems, the weakest link will generally be the signal propagation environment. Ranging accuracy is thus limited by non-line-of-sight reception and multipath interference.
Similarly, the performance of signal-strength-based positioning depends on the extent of the calibration process and the degree to which the signal propagation environment changes over time.
To continue the pace of improvement, researchers must be able to better characterize the signal propagation characteristics and incorporate this within the positioning algorithm.
Three-Dimensional Mapping
Digital road maps have been used for car navigation since the 1990s, while terrain-referenced navigation (TRN) for aircraft, which uses terrain height information, dates back even further.
Today, 3D city mapping has the potential to revolutionize positioning in challenging urban areas. Adding height information to street maps can be used to aid GNSS positioning for land vehicle and pedestrian navigation, reducing the number of satellite signals required or providing additional information to support consistency-based non-line-of-sight (NLOS) and multipath detection.
3D building information can help predict blockage and reflection of GNSS and other radio signals. This information can be used to select the most accurate ranging measurements from those available for computation of the position solution.
In principle, 3D building models can even be used to correct the errors introduced by NLOS reception and multipath interference. However, this is computationally intensive, and multipath correction requires centimeter-level building modelling to determine the signal phase shifts.
The shadow-matching technique developed by University College London (UCL) Space Geodesy and Navigation Laboratory (SGNL) uses 3D city models to predict which signals are blocked by the buildings and then compares this with the measured signal availability to determine the position. Shadow matching can be used to determine the correct side of the street in urban environments where conventional GNSS positioning is not accurate enough. Recently, it has been shown to work in real time on a consumer smartphone.
More detailed mapping is enabling map-matching techniques to be extended from vehicle to pedestrian navigation. In urban environments, trajectories can be aligned with the street grid and “snapped to” sidewalks and crossings. Building shells from city models enable indoor pedestrian trajectories to be aligned with the cardinal directions of the corridors, while full indoor mapping enables more sophisticated correction of the navigation solution.
The Multisensor Navigation Jigsaw
Historically, “integrated navigation” has typically meant the combination of two systems, such as GNSS and inertial navigation, or occasionally three, such as GNSS, odometry, and map matching. However, future integrated navigation systems are likely to have many more components.
There are two reasons for this. First, no single technology works particularly well for pedestrian navigation and other challenging applications; so, the wider the range of technologies deployed, the better the performance will be. Similarly, applications that demand high reliability will require backups to GNSS to mitigate the risk of jamming or interference.
The second driver is availability. For example, a typical smartphone contains a camera, inertial and magnetic sensors, mapping, a GNSS receiver, a Wi-Fi transceiver and the phone itself, all of which could potentially be used for navigation. In the near future, cars are likely to come with just as wide an array of different sensors suitable for navigation.
The future of navigation is thus a multisensor one, as illustrated by Figure 1 (see inset photo, above right). However, this brings two challenges. The first is system integration: how do we extract navigation information from the various sensors, radios, and databases, most of which have been installed for other purposes and supplied by a range of companies that have little interest in either navigation or working with each other?
The second challenge is optimally combining information from the various subsystems. Many sensors exhibit biases and other systematic errors that can be calibrated in an integrated system. However, subsystems can also output erroneous information due to non-line-of-sight signal propagation, misidentifying signals or environmental features, or misinterpreting host behavior. The multisensor system must detect this faulty information and prevent it from contaminating the calibration of the other sensors.
Furthermore, the error characteristics of some of the subsystems may not be completely known. Finally, the performance of different subsystems can depend on both the environment and the dynamic behavior of the host vehicle or human user, which raises the issue of context.
Context Is All
A navigation system operates in a particular context, a physical environment with a host vehicle or user that behaves in a certain way. Context can contribute additional information to the navigation solution. For example, cars remain on the road, effectively removing one dimension from the position solution. Their wheels also impose constraints on the way they can move, reducing the number of inertial sensors required to measure their motion. Similarly, pedestrian dead reckoning (PDR) using step detection depends inherently on the characteristics of human walking.
The environment affects the types of signals available. For example, GNSS reception is poor indoors while Wi-Fi signals are not available in rural areas, and most radio signals do not propagate underwater. Pedestrian and vehicle behavior also depend on the environment. A car typically travels more slowly, stops more, and turns more in cities than on the highway.
With increasing frequency, navigation systems are using contextual information, such as motion constraints, to improve performance. However, problems occur when the assumed and actual contexts diverge.
Historically, navigation systems were designed for one type of vehicle operating in a particular environment. But demand is growing for navigation systems that can operate in a variety of different contexts. The smartphone, for example, moves between indoor and outdoor environments and can be stationary, on a pedestrian, or in a vehicle.
Context-adaptive, or cognitive positioning, represents a trend just starting to emerge whereby a navigation system detects its operating context and reconfigures its algorithms accordingly. Figure 2 illustrates the concept.
Different types of environments can be distinguished based on the strengths of various classes of radio signal and the directions from which GNSS signals are receivable. Vehicle types can be identified from their velocity and acceleration profiles and by vibration signatures derived from phenomena such as engine vibration, air turbulence, sea-state motion, and road-surface irregularity.
Based on a navigation system’s detection of changes in context, its operation may adapt by processing inertial sensor data in different ways, the selection of different map-matching algorithms, and varying the tuning of the integration algorithms.
A recent example of context-adaptive positioning is pedestrian dead reckoning (PDR) algorithms that use step detection. These can determine a positioning sensor’s location on the body (e.g., hand or belt) as well as whether the person is walking or running, and then change the coefficients of the step length estimation model accordingly. Another example is a “cognitive” GNSS receiver that adapts to the quality of the signals received.
Opportunistic Navigation
The conventional approach to navigation defines a set of performance requirements and then deploys whatever infrastructure and user equipment is necessary to meet those requirements. Opportunistic navigation turns that on its head. It asks what information is already available in the surrounding environment and how it can be used to obtain the best possible position solution. Essentially, that’s how people and animals navigate when they don’t have the benefit of technology to help them.
Radio signals of opportunity (SOOP) are those intended for non-positioning purposes that are exploited for positioning without the cooperation of the operator. Examples include phone signals from a network to which one does not subscribe, Wi-Fi signals leaking from a nearby building, and broadcast TV and radio signals.
However, opportunistic information can be any measurable feature of the environment that varies spatially but not significantly over time, such as buildings and signs, magnetic anomalies, and terrain height variation. UCL SGNL is currently investigating the feasibility of similarly exploiting a number of other features, such as road texture, microclimate, sounds, and odors.
Opportunistic navigation requires a feature database to work. Maps of Wi-Fi access point locations and phone signal strengths are already commercially available for many cities, but users can build their own databases as well.
One approach to opportunistic navigation collects signal and environmental feature data whenever a GNSS position solution is available and then uses that data for positioning when GNSS is subject to interference or jamming.
Another approach, known as simultaneous location and mapping (SLAM), attempts to map the environment and determine the user position simultaneously. SLAM uses dead reckoning to measure the relative motion of the user equipment through the environment.
Cooperative Positioning
Also known as collaborative or peer-to-peer positioning, cooperative positioning occurs when a group of users work together to determine their positions and incorporates the concepts of data sharing and relative positioning.
Data sharing is the exchange of information about the surrounding environment. This can include GNSS ephemeris and satellite clock data, positions, timing offsets, and signal identification information for terrestrial radio transmitters, environmental feature data, and mapping information.
Cooperative positioning can significantly enhance opportunistic navigation. For example, two peers at known positions using cameras to observe an unknown landmark can determine its position and send this information to a third peer, who can then use the landmark to help determine his or her own position.
Data sharing can be used by itself. However, a full cooperative positioning system also incorporates relative positioning, where users measure and share the range between them or similar information. This makes use of signals and features that others (peers) have observed to help determine position and is particularly useful for users who can’t achieve a stand-alone position solution.
Integrity
As technologies mature, users expect greater reliability. In turn, more reliable technology is trusted for use in safety-critical and mission-critical applications. Over the past two decades or so, a rigorous integrity framework has been established that enables GPS to meet the demanding safety requirements of civil aviation. Other applications are waiting in the wings for high integrity positioning, such as shipping, advanced rail signalling, location-based charging schemes, and virtual security fences.
However, for most of these applications, no single positioning technology works reliably across all environments. Consequently, the challenge to the navigation community is to produce a multisensor integrated navigation solution that can meet demanding integrity requirements even when its constituent systems are unreliable.
Bringing It All Together
Figure 3 shows how the concepts discussed in this article could be brought together as an integrated navigation system. Many of these ideas will mature over the next ten or so years, while others may fall by the wayside and new ideas emerge to take their place.
To meet some of the greatest positioning challenges of our times — reliable pedestrian navigation, lane identification, and robustness against interference, jamming and spoofing — we need to bring these various ideas together.
The next generation of integrated navigation systems will inevitably be more complex than the current generation. Consequently, the navigation and positioning community must find new ways of working together so that this technology can reach its true potential.
Additional Resources
[1] Abdulrahim, K., and C. Hide, T. Moore, and C. Hill, “Aiding Low Cost Inertial Navigation with Building Heading for Pedestrian Navigation,” Journal of Navigation, Vol. 64, No. 2, 2011, pp. 219-233
[2] Brown, D., and L. Mauser, B. Young, M. Kasevich, H. F. Rice, and V. Benischek, “Atom Interferometric Gravity Sensor System,” Proceedings of IEEE/ION PLANS 2012
[3] De Agostino, M., “A Multi-frequency Filtering Procedure for Inertial Navigation,” Proceedings of IEEE/ION PLANS 2008
[4] Faragher, R. M., C. Sarno, and M. Newman, “Opportunistic Radio SLAM for Indoor Navigation using Smartphone Sensors,” Proceedings of IEEE/ION PLANS 2012
[5] Garello, R., and L. Lo Presti, G. E. Corazza, and J. Samson, “Peer-to-Peer Cooperative Positioning, Part 1: GNSS-Aided Acquisition,” Inside GNSS, March/April 2012
[6] Garello, R., and J. Sanson, M. A. Spirito, and H. Wymeersch, “Peer-to-Peer Cooperative Positioning, Part II: Hybrid Devices with GNSS & Terrestrial Ranging Capability,” Inside GNSS, July/August 2012
[7] Grejner-Brzezinska, D. A., et al., “Challenged Positions: Dynamic Sensor Network, Distributed GPS Aperture, and Inter-nodal Ranging Signals,” GPS World, September 2010
[8] Groves, P. D., Principles of GNSS, Inertial, and Multisensor Integrated Navigation Systems, Second Edition, Artech House, Boston, MA, 2013
[9] Groves, P. D., et al., “Intelligent Urban Positioning using Multi-Constellation GNSS with 3D Mapping and NLOS Signal Detection,” Proceedings of ION GNSS 2012
[10] Hide, C., T. Botterill, and M. Andreotti, “An Integrated IMU, GNSS and Image Recognition Sensor for Pedestrian Navigation,” Proceedings of ION GNSS 2009
[11] Kalliola, K., “High Accuracy Indoor Positioning Based on BLE,” Nokia Research Center Presentation, April 27, 2011
[12] Lin, T., C. O’Driscoll, and G. Lachapelle, “Development of a Context-Aware Vector-Based High-Sensitivity GNSS Software Receiver,” Proceedings of ION ITM 2011
[13] Pei, L., et al., “Using Motion-Awareness for the 3D Indoor Personal Navigation on a Smartphone,” Proceedings of ION ITM 2011
[14] Robertson, P., M. Garcia Puyol, and M. Angermann, “Collaborative Pedestrian Mapping of Buildings Using Inertial Sensors and FootSLAM,” Proceedings of ION ITM 2011
[15] Shafiee, M., K., O’Keefe, and G. Lachapelle, “Context-aware Adaptive Extended Kalman Filtering Using Wi-Fi Signals for GPS Navigation,” Proceedings of ION GNSS 2011
[16] Shivaramaiah, N. C., and A. G. Dempster, “Cognitive GNSS Receiver Design: Concept and Challenges,” Proc. ION GNSS 2011.
[17] Shkel, A. M., “Microtechnology Comes of Age,” GPS World, September 2011.
[18] Veth, M. J., Fusion of Imaging and Inertial Sensors for Navigation, Ph.D. dissertation, Air Force Institute of Technology, 2006.
[19] Wang, L., and P. D. Groves and M. K. Ziebart, “GNSS Shadow Matching: Improving Urban Positioning Accuracy Using a 3D City Model with Optimized Visibility Prediction Scoring,” Proceedings ION GNSS 2012, Institute of Navigation, Nashville, Tennessee USA, September 2012
[20] Yuksel, Y., and N. El-Sheimy, and A. Noureldin, “Error Modeling and Characterization of Environmental Effects for Low Cost Inertial MEMS Units,” Proceedings of IEEE/ION PLANS, 2010