With a collection of some of the best researchers in space and aeronautics involved, the sky may indeed just be the limit.
The European Space Agency (ESA) and Stanford University are challenging global artificial intelligence (AI) specialists to train software to judge the position and orientation of a drifting satellite with a single glance. Such a skill could be used in the future for servicing or salvaging spacecraft, according to the ESA.
ESA’s Advanced Concepts Team (ACT) has teamed up with Stanford University’s Space Rendezvous Laboratory (SLAB) for its latest competition to harness machine learning for space-related goals.
The topic of the competition is satellite “pose estimation”: identifying the relative position and pointing direction (known as attitude) of a target satellite from single snapshots of it moving through space.
“Achieving pose estimation of uncooperative targets will play an important role in future satellite servicing, enabling refurbishment of expensive space assets,” explains Prof. Simone D’Amico, the lab’s founder.
“It is also key to the debris removal technologies required to ensure humankind’s continued access to space as well as the development of space depots to facilitate travel towards more distant destinations.”
To ensure maximum realism, the competition is based on results from an actual space mission. PRISMA – launched by the Swedish Space Corporation with the support of the German Aerospace Center, the Technical University of Denmark and French space agency CNES – was an experimental two-satellite mission to test formation-flying and rendezvous techniques, launched in 2010.
“The two PRISMA small satellites, Tango and Mango, took multiple photos of one another over the course of the mission,” said Dario Izzo of ESA’s ACT, which oversees the competition.
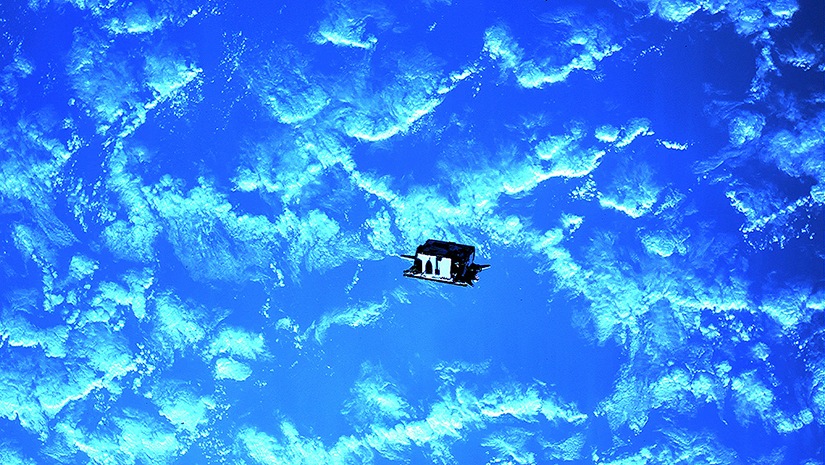
“There weren’t enough of these snapshots to train algorithms with, but Prof. D’Amico’s PhD student Sumant Sharma at Stanford’s Space Rendezvous Laboratory has generated fully realistic equivalents. These are a combination of fully digital images and physical photos taken using satellite models in representative lighting conditions as part of his research.
“We will supply teams with around 15,000 synthetic images plus another 1,000 actual images. Some 80% of them will come with pose data included, which can be used for machine learning. The aim of the competition is to estimate the satellite pose as accurately as possible, and submissions will be evaluated on the other 20% for which pose data is not released.”
ACT Young Graduate Trainee Mate Kisantal adds, “Using a mix of real and digital imagery for evaluation helps to add greater certainty that such a solution would actually work in space. Algorithms trained on digital imagery only can sometimes experience a ‘reality gap’ where they find it hard to transfer their learning to real-world views.
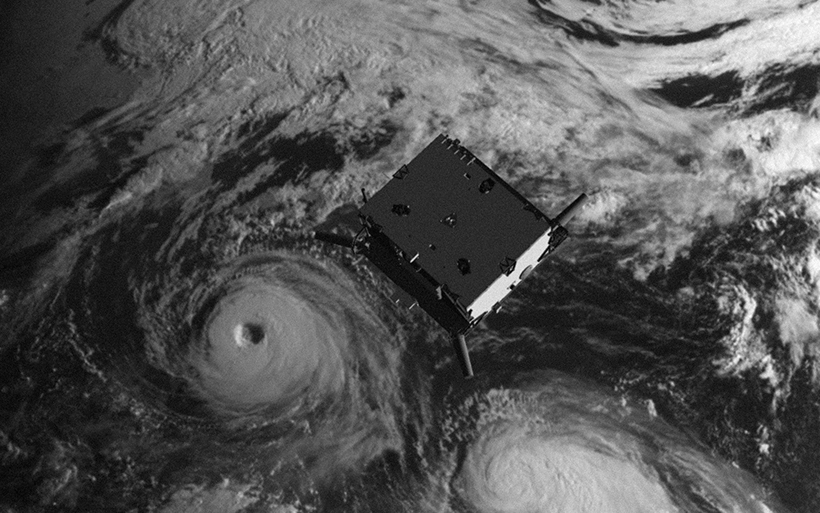
“The grayscale images show a variety of different attitudes and distances – from between 5 to 40 meters away – and different lighting conditions. Some have a realistic Earth in the background, others have the blackness of space. Some blurring and white noise have also been added.”
Prof. D’Amico has direct experience of PRISMA, having worked on its relative navigation and control systems and serving as its Principal Investigator for DLR.
“Machine learning algorithms for aerospace applications require rigorous, well understood training data,” he said. “PRISMA’s rich space imagery and associated flight dynamics products offer that combination – although no proprietary data from the mission is being disclosed.
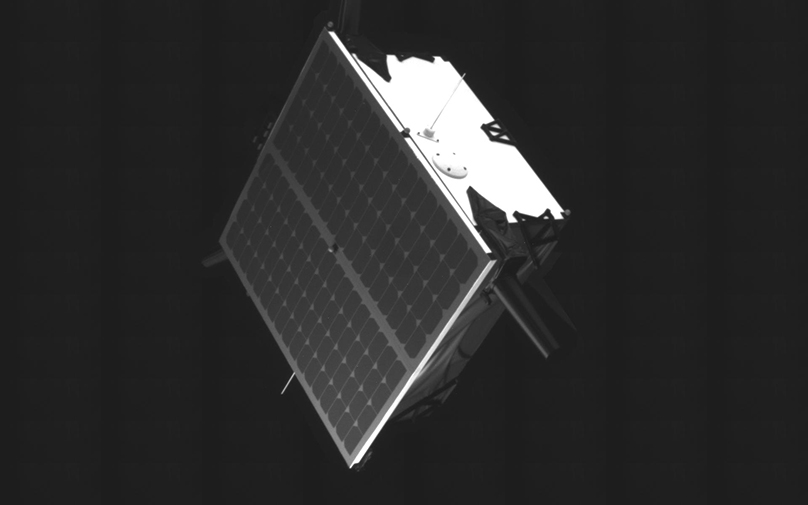
“By making this massive, rigorously labelled dataset available to the machine learning community, we hope to engage them in an important spaceborne navigation problem, comparing potential solutions from a worldwide community.”
He sees the potential payoff as a new way of operating in space: “The very first space rendezvous happened just over 53 years ago, between Geminis 6 and 7 in December 1965, enabled by radar, a computer plus a human-in-the-loop. Success made the Moon landings possible, along with the large-scale space station construction that followed.
“But new eras come with their challenges, and methods to solve them. The aim now is to develop rendezvous and formation flying techniques for miniaturized, autonomous satellites, which could work together as distributed systems – meaning multiple small satellites working together to accomplish objectives that would be impossible for a single monolithic satellite.”
This is the latest competition hosted at the ACT’s Kelvins website, named after the temperature unit of measurement – with the idea that competitors should aim to reach the lowest possible error, as close as possible to absolute zero.
“We’ve been thinking a lot about how to apply AI to space problems, and Kelvins is an important part of that,” adds Dario. “By making all kinds of big datasets available to the wider machine learning community we can see what they get out of them, and potentially derive some valuable new approaches.”
Photos and background for this article came from the ESA. For more information, click here to read a story on the Stanford Engineering website: https://stanford.io/2Bz4CH2