The HAPS Augmented Search-And-Rescue Demonstration System (HASARDS) project could provide localization, tracking, georeferenced imagery and communication relay, complementing the existing system to help better execute SARs missions.
Elie Amani, Benjamin Queval, Jérémy Skelton, Avag Tsaturyan, Sofien Baklouti, M3 Systems
Florin-Catalin Grec, ESA, and Etienne Bargeton, Stratos Solution
Since the beginning of the 1980s, satellite-based search and rescue (SAR) activities have become widespread, enabling alert detection from the vantage point of Earth orbits. SAR consists of three main and interdependent activities: detecting emergency alerts, locating, and rescuing people and vessels, airplanes, etc., in distress.
Cosmicheskaya Systyema Poiska Aariynyich Sudov (COSPAS), which translates to Space System for the Search of Distressed Vessels and Search & Rescue Satellite Aided Tracking System (SARSAT) payloads are provided by the United States, the Russian Federation, India and the European Organization as part of an international cooperative satellite-based radiolocation system to support SAR operations for aviators, mariners and land travellers in distress. The system comprises low Earth orbiting (LEO), medium Earth orbiting (MEO) and geostationary Earth orbiting (GEO) satellites.
Figure 1 depicts the COSPAS-SARSAT (C/S) system overview. It illustrates the sequence of events starting from the moment an emergency beacon is activated up to the launch of SAR assets toward the distress location.
With the use of GNSS constellations in MEO for SAR (MEOSAR) in addition to LEOSAR and GEOSAR, multiple satellites are visible to an activated beacon. This provides instantaneous detection and fast localization of an alert. The pursued objective is to eliminate the “search” element from SAR activities, or at least considerably reduce its duration.
Nevertheless, for some emergencies, even with the extensive view provided by MEOSAR, complementary means are still needed to augment and significantly improve SAR capabilities. The HASARDS project, a joint project between Stratos Solution, M3 Systems and Sonaca, was intended to explore the complementary, augmentative solutions a HAPS system could offer to the SAR community. The HASARDS project was funded by the European Space Agency (ESA) as part of its “NAVISP Element 1” program. HASARDS stands for HAPS-Augmented Search-And-Rescue Demonstration System.
Improving Contextual Information
The main goal of HASARDS is to use High Altitude Pseudo Satellites (HAPS) to bring augmentation and additional features to the SAR system. From SAR actor activity analysis, the objectives of a HAPS layer as complement to the existing C/S would be to support situational awareness, decision-making, planning, coordination, and execution of SAR missions. In this perspective, one could classify user needs into the following three categories:
1. Detect Distress Situation (DS):
• Detect and get notified upon new situations.
• Detect and get notified upon significant updates.
2. Build up consolidated information about the distress situation:
• DS Localization: i.e., WHERE and WHEN the distress situation is.
• DS context: The WHO or WHAT (given by the beacon type and the beacon ID, or by HAPS imagery).
3. Communicate to support information sharing, coordination and execution of the SAR mission (HOW)
• To improve awareness on WHAT the distress situation is (context).
• To improve the HOW: Coordinate and execute the mission.
The potential improvements of the HAPS layer over terrestrial systems are wider coverage and less interference. In comparison with C/S satellites, HAPS offers lower latency, better radio link budget, quasi-static motion, the possibility of payload reconfiguration or maintenance, and direct georeferencing. Figure 2 depicts the COSPAS/SARSAT system with a HAPS layer.
The main objective of HASARDS is to bring value to SAR systems. From SAR actor activity analysis, the objectives of HASARDS in complement of the existing C/S would be to support:
• Situational awareness
• Decision making
• Planning
• Coordination and execution of the SAR mission
Favoring a light coupling with the existing C/S system to avoid complexity, the promoted solution features independent, HAPS-based signal localization in conjunction with direct georeferencing. Additional options were proposed, such as AIS/VDES signal detection, implementation of HAPS-specific Return Link Message (RLM), and GSM (cell phone) airborne relay.
A HAPS layer MATLAB simulator has been developed by M3 Systems, in the context of the HASARDS project, for a preliminary assessment of localization and tracking benefit, considering the multilateration of the C/S beacon signals georeferencing technique and GNSS position or other.
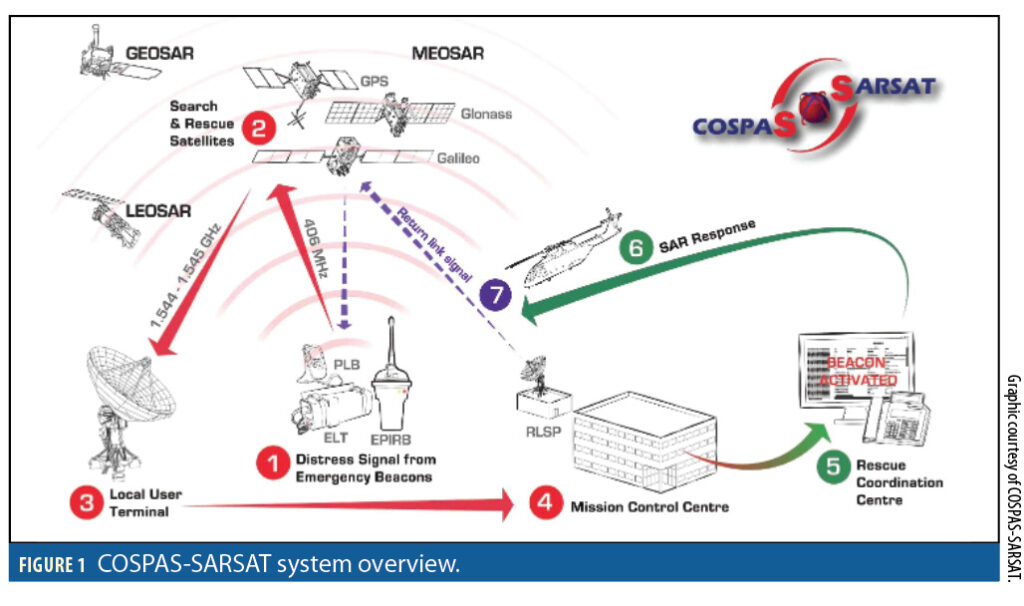
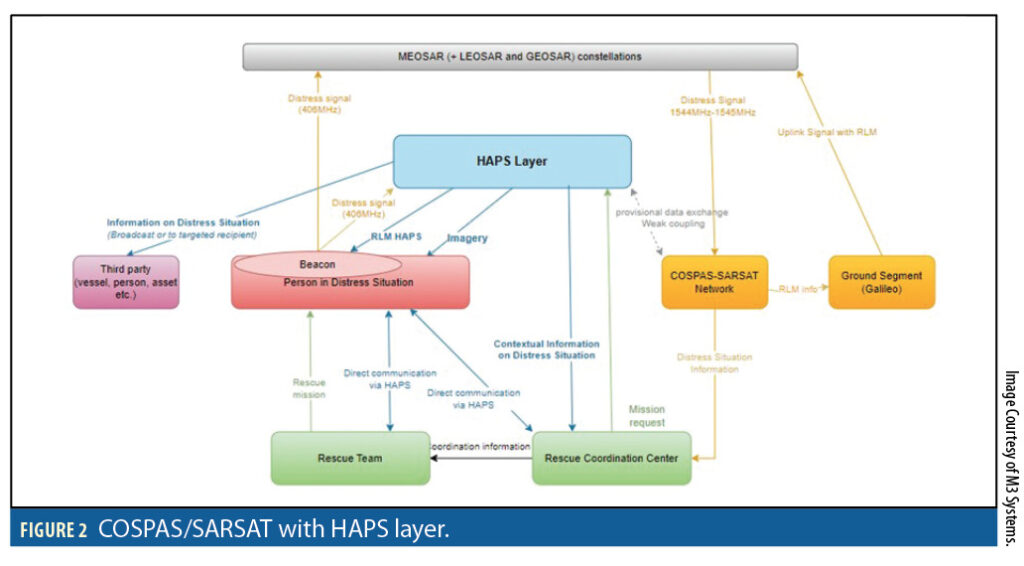
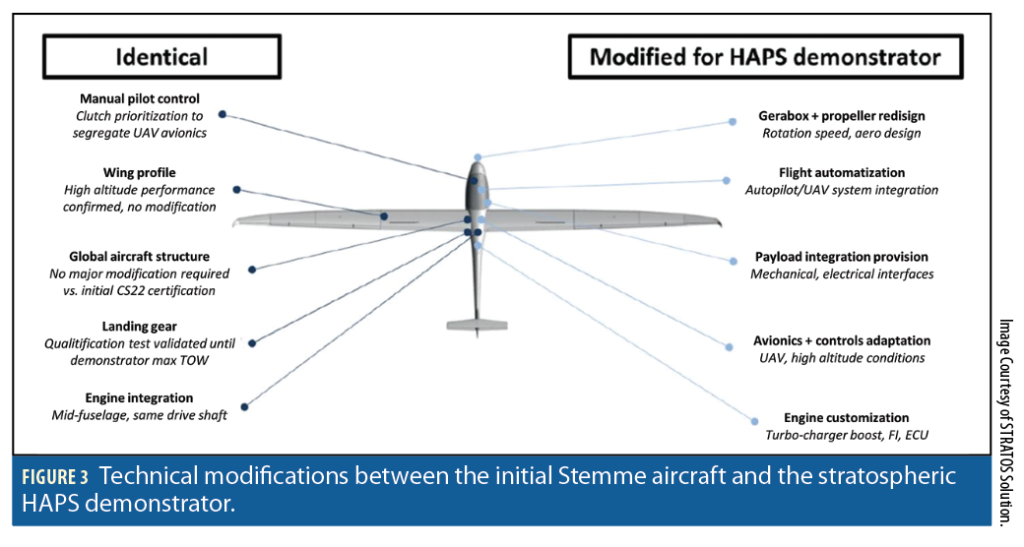
The HAPS
HAPS technical knowledge and related Concept of Operations (CONOPS) were brought by Stratos Solution thanks to its experience developing a HAPS prototype. This prototype, developed in partnership with Sonaca and Stemme, is a fixed-wing HAPS demonstrator developed from a Stemme aircraft basis by adapting it to stratospheric conditions.
The technical modifications that transformed an existing Stemme aircraft into a UAV demonstrator are illustrated in Figure 3.
Stratos Solution leveraged its expertise in Earth observation operations to assess the expected performances of a HAPS-based direct georeferencing solution using high-end GNSS/IMU equipment. The precise knowledge of the camera orientation and the elevation map makes it possible to estimate the target’s position. At 100 km, the RMS errors are to the order of 1 km and 100 m in longitudinal and transverse directions respectively (with respect to HAPS), and mostly depends on the IMU performances.
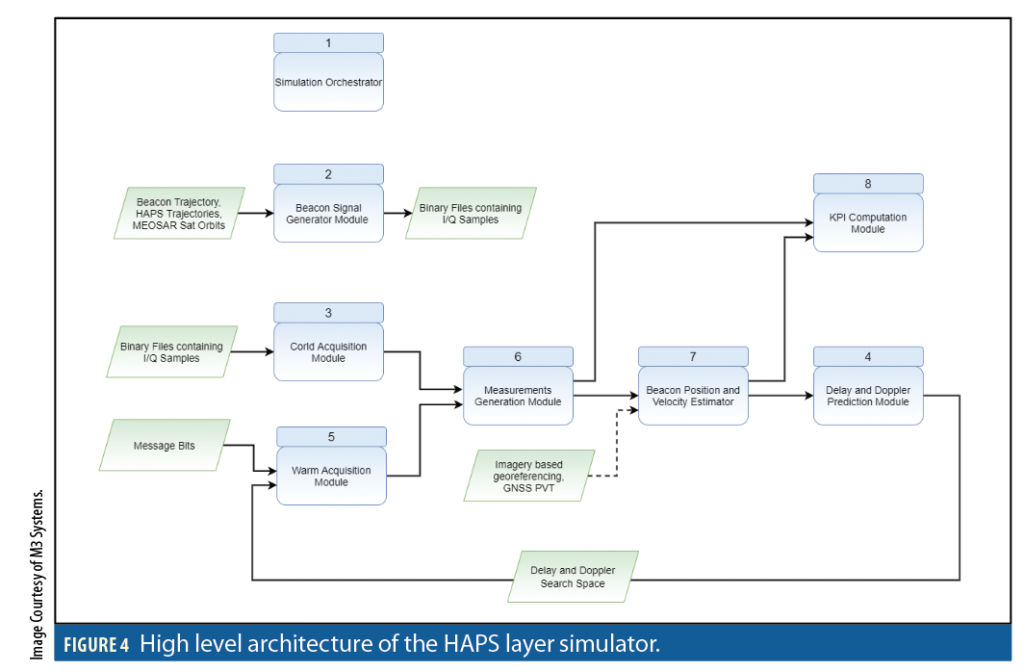
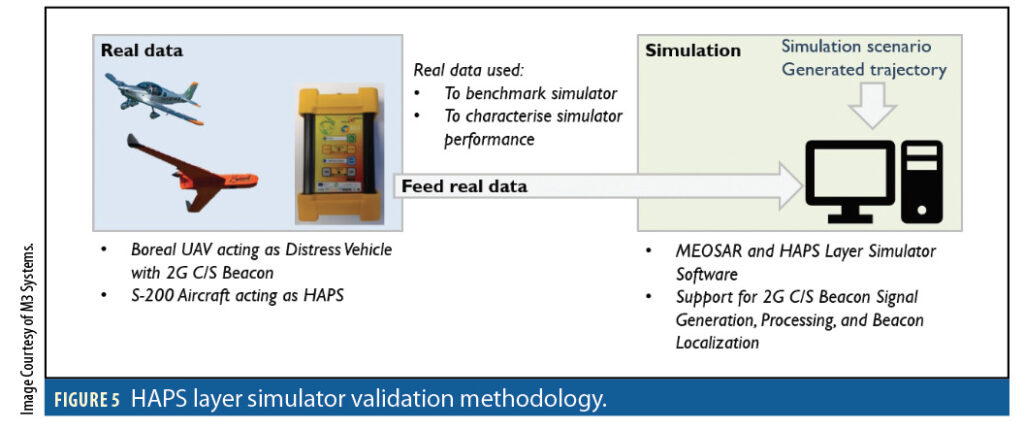
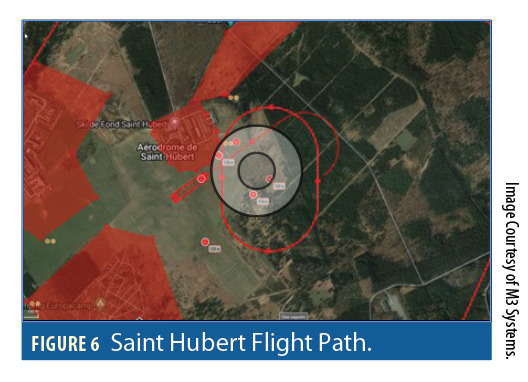
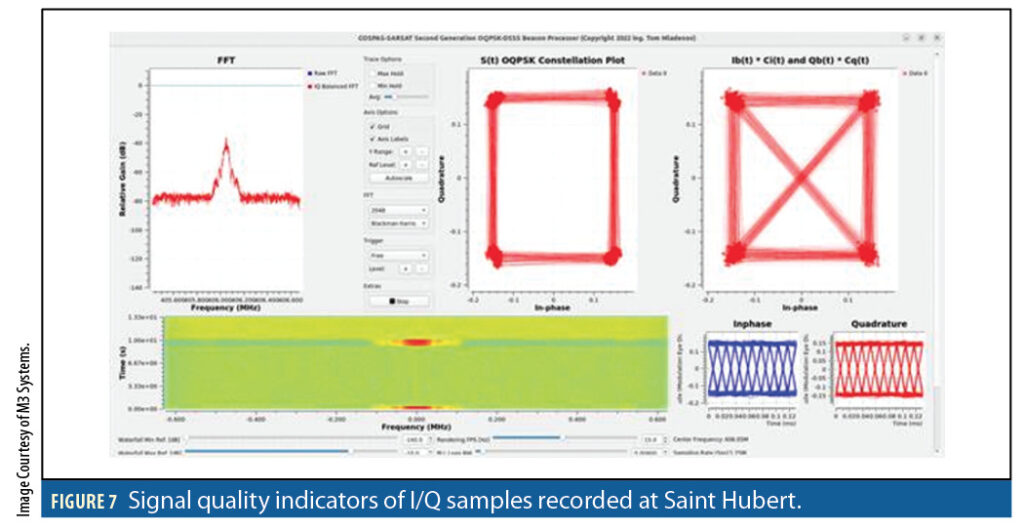
The HAPS Layer Simulator
The purpose of the simulator is to evaluate the performance of the HAPS layer and the added value with respect to the existing C/S system. The simulator, illustrated in Figure 4, is composed of the following modules: simulation orchestrator, beacon digital signal generator, cold acquisition module, warm acquisition module, raw measurements generation module, beacon position and velocity estimation module, delay/Doppler prediction module, and a key performance indicator (KPI) computation module. The simulator incorporates the HAPS layer as well as MEOSAR. The beacon signal is generated first, in the form of digital in-phase/quadrature (I/Q) samples, then processed by the simulator for beacon localization. The simulator’s main features are:
• The beacon signal generator uses HAPS/MEOSAR orbits and beacon trajectory as inputs to generate a 406 MHz 2G beacon signal (digital I/Q samples) compliant with C/S T.018 specifications document.
• The simulator performs beacon signal detection (acquisition) using an FFT-based approach, time of arrival (TOA) and frequency of arrival (FOA) measurements formation, beacon position and velocity estimation using an unscented Kalman filter, delay and Doppler prediction to facilitate warm beacon signal acquisition after the first beacon position fix, and relevant KPI computation.
The simulator inputs are:
• I/Q binary file containing beacon signal (digital I/Q samples) as received from HAPS or MEOSAR.
• HAPS orbits (timestamped positions and velocities).
• MEOSAR satellites orbits (timestamped positions and velocities).
• The I/Q binary file containing beacon signal (digital I/Q samples) used as input to the simulator is either synthetically generated by the beacon signal generator or the result of an I/Q signal recording campaign using an I/Q signal recorder.
The following hypotheses/assumptions were taken for the beacon digital signal (I/Q samples) generator:
• The generated beacon digital signal is the down-converted and digitized 406 MHz beacon signal after reception at the available HAPS constellation and the available MEOSAR satellites constellation.
• The distance between MEOSAR and MEOLUTs or MEOSAR and MCC is known or can be obtained knowing MEOSAR orbits and MEOLUTs/MCC positions. The main unknown in the beacon signal travel time from beacon to MEOLUTs/MCC is the travel time from beacon to MEOSAR/HAPS. Therefore, once measured, it can be used as a TOA measurement.
• The TOA measurements (pseudoranges) and FOA measurements (Doppler) estimated by measuring code delays and Doppler via acquisition/refinement of the generated beacon digital signal are representative of reality and are sufficient to estimate beacon position and velocity.
The following hypotheses/assumptions were made for the beacon acquisition/tracking/position computation simulator:
• The simulator does not include MEOLUTs (LUT: Local User Terminal) and MCC.
• Therefore, the simulator does not cover communication between some C/S system components (MEOSAR to MEOLUTs, MEOLUTs to MCC, etc.).
• Abstraction from these components is not a hindrance toward the simulator results representativeness with respect to the real COSPAS/SARSAT system.
The quality of the signal produced by the signal generator (spectrum, OQPSK constellation plot, eye diagram, and time domain signal) was validated using data recorded during field experiments. Digital I/Q samples recorded on field (Saint Hubert airdrome) using a M3 Systems I/Q recorder were compared with digital I/Q samples produced by the signal generator using HAPS orbits and beacon trajectory from the same field experiment. Figure 5 illustrates the simulator validation methodology.
Experimental and Simulation Results
Figure 6 depicts the flight path during the field experiment at Saint Hubert airdrome. Figure 7 (from « GNU Radio OQPSK-DSSS Second Generation SAR IQ Processor » tool, developed by Tom Mladenov) shows signal quality indicators (spectrum, OQPSK constellation plot, eye diagram, and time domain signal) for the recorded I/Q samples during that experiment. These were used to validate the developed simulator, especially beacon signal generation.
Figures 8 and 9 show how using the GNSS beacon position that is encoded in the beacon message can improve beacon positioning if this information is used to initialize the unscented Kalman filter. The filter convergence to the beacon position is faster.
Figures 10 and 11 illustrate the impact of using georeferencing information as assistance to beacon positioning. Convergence and accuracy of the calculated beacon position are improved. This, of course, depends on the accuracy of the used georeferencing information.
Table 1 provides C/S MEOLUT performance specifications for different beacon dynamics (nearly static, medium speed and fast). These are used as design guidelines for beacon localization systems. Table 2 provides a summary of beacon localization performance for different simulated scenarios. The MEOSAR scenario that is presented as reference only made use of 4 MEOSAR satellites. The idea is to compare performance between a scenario using four MEOSAR and a scenario using four HAPS for beacon localization. Following the guidelines in Table 1, the percentage of locations within 500 m, 1 km, 5 km, and 7 km is computed, within 5 minutes from the first beacon message transmission. The obtained results for four MEOSAR and slow moving beacon, four HAPS and slow moving beacon, four HAPS and slow moving beacon (with GNSS), four HAPS and slow moving beacon (with georeferencing), four HAPS and fast moving beacon, and four HAPS and fast moving beacon (with GNSS), are provided in Table 2.
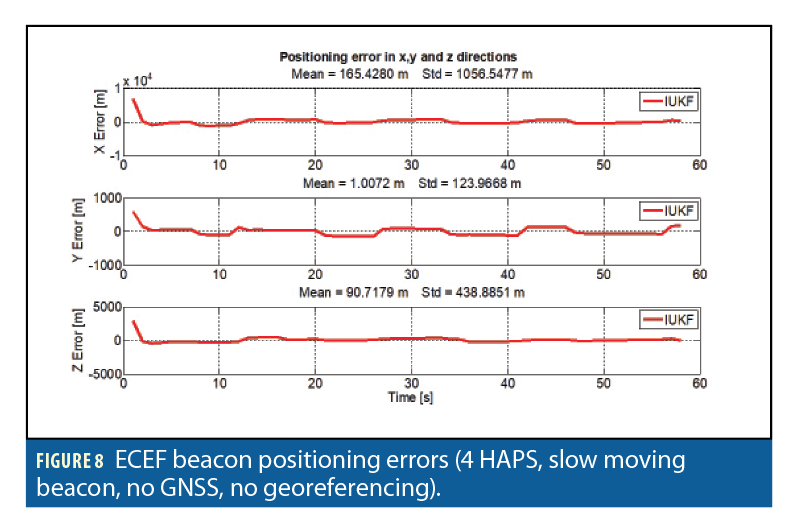
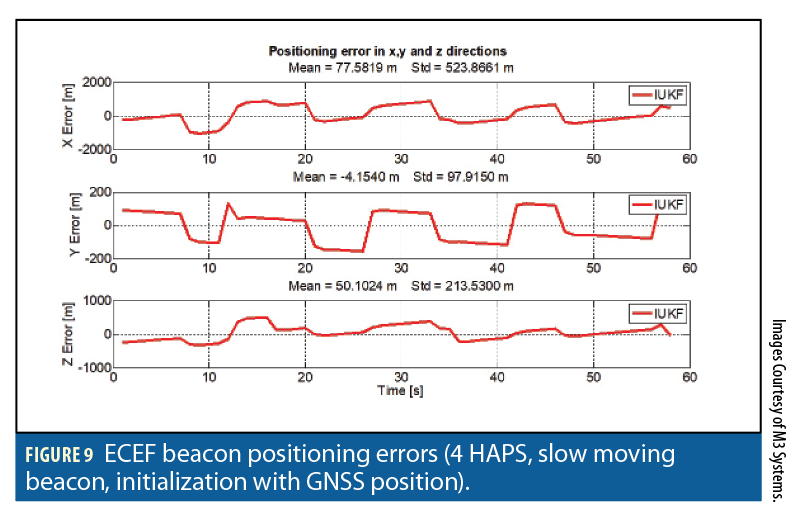
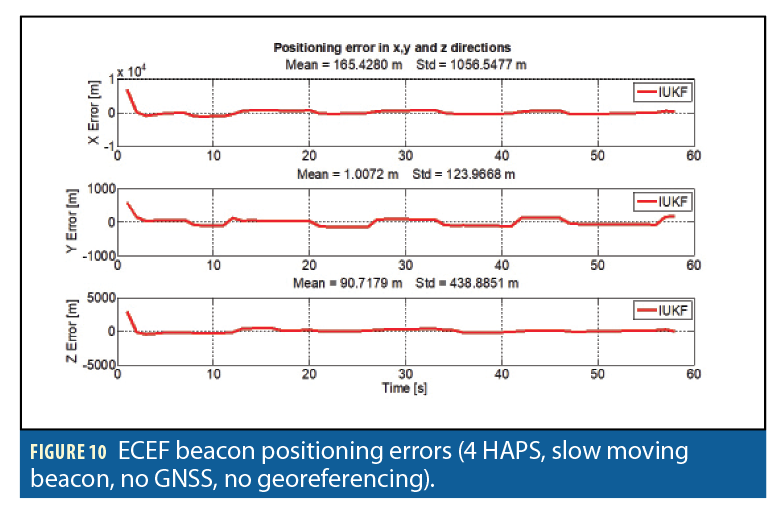
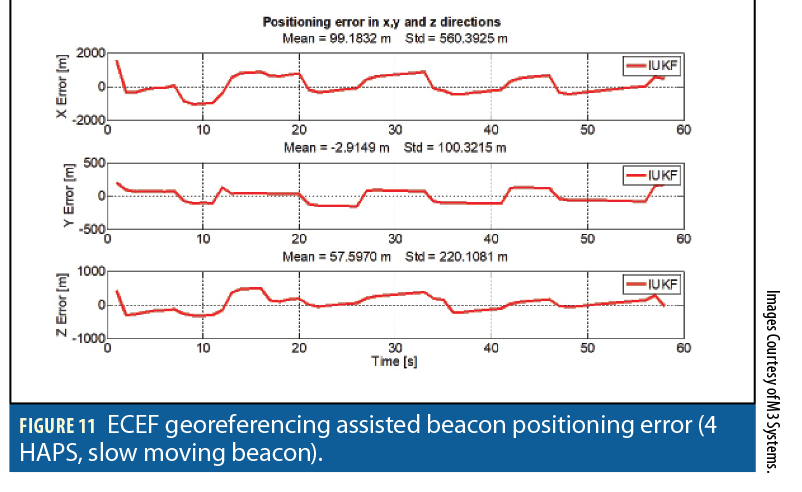
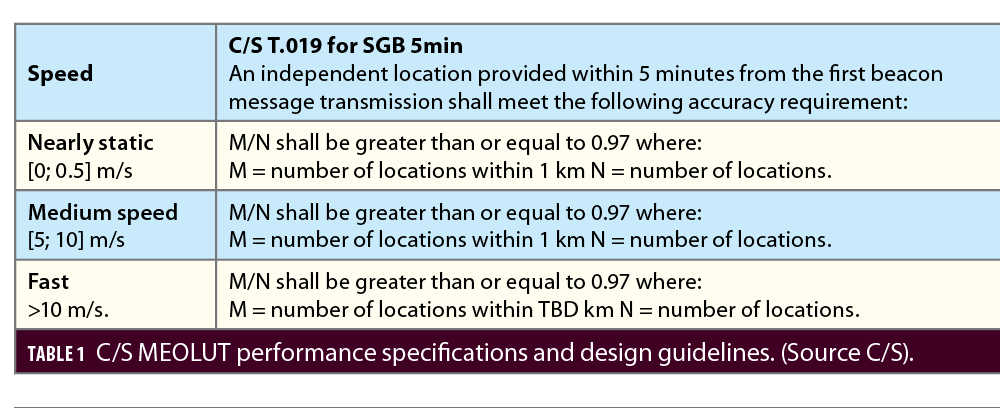
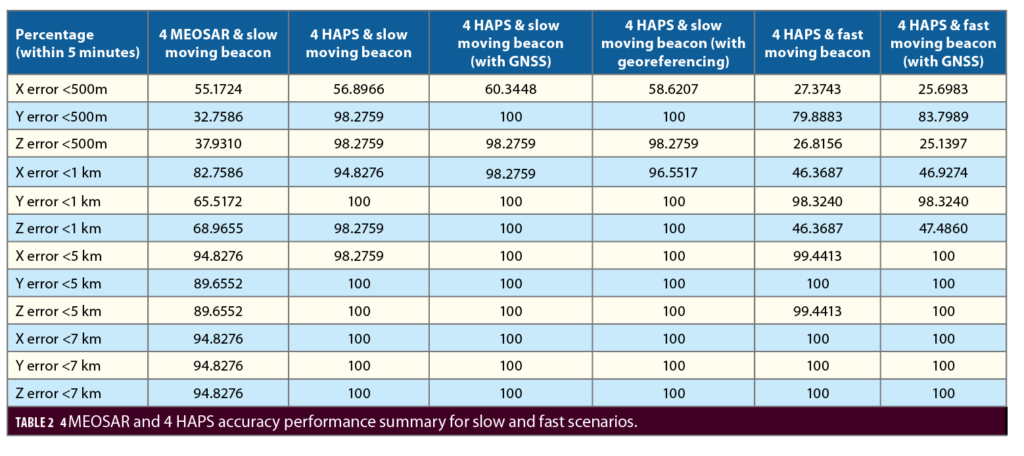
Conclusion
Dynamic simulation results confirm that a HAPS layer can locate a distress situation with an error (standard deviation) of less than 500 m with four HAPS based operations. With static cases, the mean absolute positioning error is less than 170 m and the standard deviation less than 1.1 km. For tracking fast objects, the mean absolute positioning error is less than 40 m and the standard deviation less than 1.8 km, which would comply with GADSS ICAO CONOPS requirements of having an estimation of the aircraft crash within a 6 NM radius.
In all cases, the initialization with GNSS information would help the localization algorithm converge faster and provide more accurate results. The calculated position accuracy improves in comparison with the scenario that does not use GNSS information (mean absolute value below 80 m and standard deviation below 550 m).
The georeferencing is promising in terms of performance as it has an error of the same order of magnitude as the TOA/FOA algorithm when the target is on at ground or sea level and provides more accurate results when the HAPS is around 110 km from the beacon or less, in the case of a static distress vehicle at least. Georeferencing is very dependent on distress vehicle height, which can introduce significant error bias due to perspective projection.
The HASARDS project promoted a C/S independent HAPS layer system solution. Such a system could bring high value to SAR operations in terms of localization, tracking, imagery for contextual information, and communication relay. The communication brick would take advantage of HAPS payload diversity, such as 4G/5G, AIS/VDES, to connect the SAR system, SAR team, other third parties and persons in distress.
More specifically, the HASARDS project showed that georeferenced imagery could deliver great localization performance. This solution could be:
• Used in conjunction with multilateration of the radio beacon signal to provide even greater performance and reduce the number of HAPSs, hence the operational costs.
• Used as a very performant shorter-range localization means with on-board detection means (C/S, AIS/VDES etc).
• Used as a very performant shorter-range localization means deployed on the distress area upon rescue coordination center (RCC) request.
The multi-mission approach (i.e., distress beacon detection and imagery) is the most promising, as the operating costs could be shared across institutional agents and governments thanks to a high level of synergy between ISR, Coast Guard and SAR applications.
The final meeting of the HASARDS project, scheduled on March 8th , will reflect on the achievements and challenges met during the project and will close these last months of work afterwards.
Authors
Elie Amani received a Ph.D. in GNSS Signal Processing from the Doctoral School of Mathematics and Information and Communication Sciences and Technologies (MSTIC) of Paris-Est Créteil University (UPEC)in 2017, with a specialization in Signal, Image and Automation. His research focused on satellite-based positioning in constrained environments using scalar and vector tracking methods with detection and exclusion of satellites contaminated by multipath from PVT calculation. He is currently a GNSS and signal processing architect and engineer at M3 Systems.
Florin-Catalin Grec holds a MSc (2015) in Geomatics from the Polytechnic University of Milano and BSc (2013) in Geodesy from the Polytechnic University of Timisoara. He is currently working as GNSS Evolutions Strategy Engineer in the Directorate of Navigation at ESA where he contributes to R&D efforts on hybrid positioning based on GNSS and telecommunication signals broadcast by 5G networks, and LEO PNT. He also contributes to 3GPP RAN1 and RAN2 working groups where he is playing a central role in the standardization of GNSS features in mobile wireless standards since 2017.
Etienne Bargeton is an aerospace engineer who graduated from ISAE-Supaero. He spent many experience-rich years at Sonaca, working in aerospace structure sizing and justification. It was during this period that he joined Sonaca’s internal innovation program, which eventually led to a HAPS demonstrator project. Strong believer of this promising technology, Etienne then joined Stratos Solution in 2021, as a project leader on HASARDS, willing to make this technology come true.
Benjamin Queval works at M3 Systems Belgium as an ATM and UAS system expert. Holding an MSc from Cranfield University and ENSAM Paristech, Benjamin has contributed to the development of numerous complex systems for manned and unmanned aviation as well as for ATM for more than 10 years.
Jérémy Skelton graduated in electrical engineering from the « Université Catholique de Louvain » (UCL, Belgium) in 2009. He is specialized in telecommunications. From 2004 to 2007, he was involved in a startup project as a software analyst. From 2010 to 2019, he was involved in numerous IT infrastructure projects as a system engineer. He has joined M3 Systems in 2019 as project leader and particularly involved in GNSS projects focused on hybridization with other sensors.
Avag Tsaturyan graduate from National Polytechnic University of Armenia in 2016 and holds master’s degree in automation and control. From 2014-2018 he worked as a Systems Engineer in National instrument Yerevan, focusing in automotive and HIL applications. He joined M3 Systems as a Systems Engineer in 2018 and supports GNSS test and measurement activities within company.
Sofien Baklouti graduated with a master’s degree in aeronautical and space systems engineering from the University of Paris-Saclay. He also held a second engineering degree in embedded computing from the National School of Electronics and Telecommunications. This allowed him to acquire a solid foundation in real-time applications for intelligent automotive systems. He joined M3 Systems as Embedded Development Engineer in 2020.