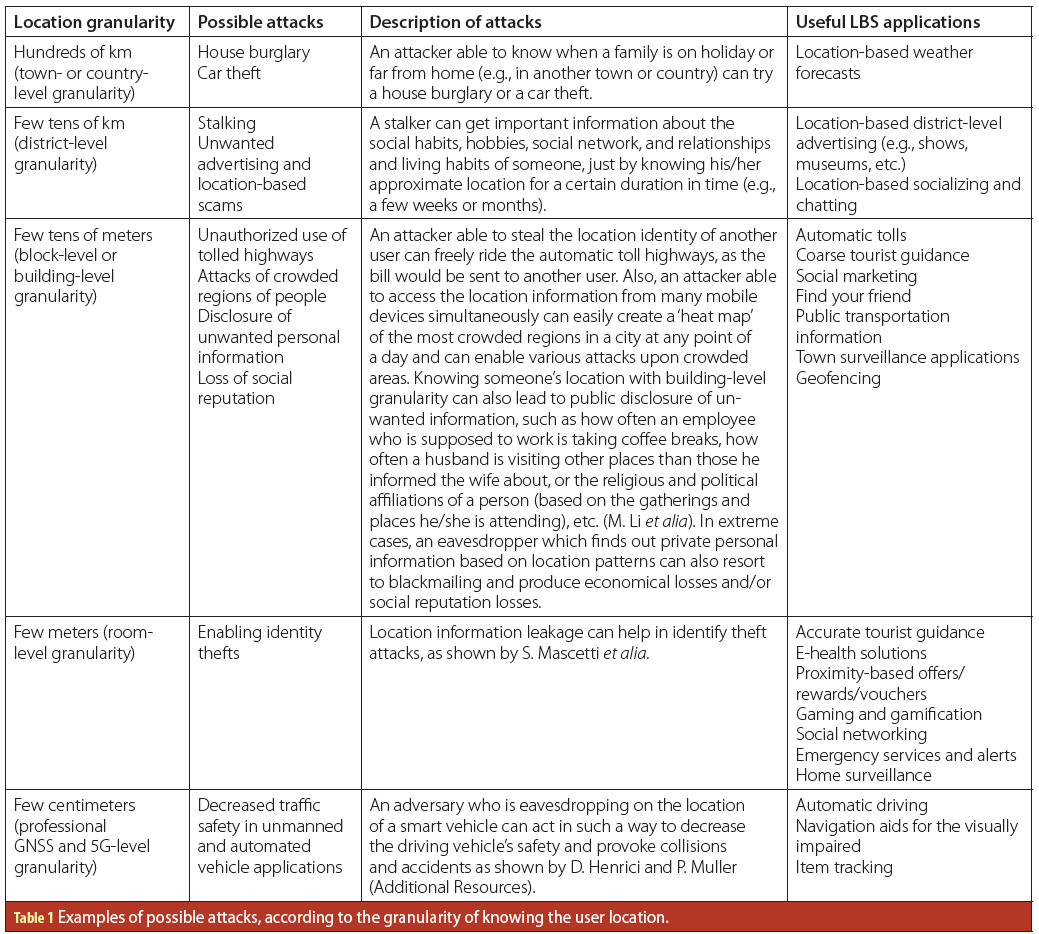
Working Papers explore the technical and scientific themes that underpin GNSS programs and applications. This regular column is coordinated by Em. Univ.-Prof. Dr.-Ing. habil. Dr. h.c. Günter W. Hein.
This is the second article in a series. For Part 1: GNSS localization, see here.
Working Papers explore the technical and scientific themes that underpin GNSS programs and applications. This regular column is coordinated by Em. Univ.-Prof. Dr.-Ing. habil. Dr. h.c. Günter W. Hein.
This is the second article in a series. For Part 1: GNSS localization, see here.
GNSS solutions are widely spread and currently able to provide excellent navigation performance under a variety of scenarios, especially with the advent of assisted and cloud GNSS solutions. However, when used indoors and in deep urban canyons, they still suffer from many challenges such as outages of the system due to very low signal powers received indoors or very high positioning errors due to multipath. There are two main ways to circumvent such problems and they are addressed in the following two sections: using hybrid solutions between GNSS and a typically complementary solution, such as cellular, wireless local area network (WLAN), or inertial sensors, or using purely non-GNSS solutions, which might be desirable, for example, on low-cost mobile devices not supporting GNSS chipsets.
Hybrid-GNSS Localization
Hybrid positioning techniques merge GNSS and non-GNSS technologies to provide an accurate position of the user or device. The non-GNSS category typically includes all the terrestrial navigation systems, ranging from cellular to WLAN, and from Ultra Wide Band (UWB) to Inertial Navigation Systems (INS).
Non-GNSS positioning signals are typically referred to as Signals of Opportunity (SoO). SoO by definition means any signal which can be used for positioning, but which, unlike GNSS, was not initially designed with positioning purposes in mind (e.g., cellular, WLAN, UWB, etc.,). A debatable category is the category of 5G cellular signals, which currently are being designed in such a way to also support positioning, and thus they no longer belong to the SoO category. We will briefly discuss 5G positioning in the next section.
Another arguable category is the category of Internet of Things (IoT) and the related IoT positioning. The IoT concept is based on the connection of sensing devices to the internet, with the objective of using the information provided by the sensors (i.e., positioning information) in different applications, such as LBS or transportation and logistics. IoT positioning sensor networks can be divided into homogenous or heterogeneous. The heterogeneity definition deserves a discussion by itself, but in here we refer to this heterogeneity as follows. In a homogenous IoT architecture, the network is only formed by either GNSS or non-GNSS sensors. In a heterogeneous IoT architecture, the network is formed by both GNSS and non-GNSS sensors, whose information is then processed by a control unit applying hybrid positioning techniques. Hybrid (or heterogeneous) IoT positioning is discussed in this section. Homogeneous IoT positioning along with modern navigation solutions purely based on non-GNSS systems are discussed in the next section. The rest of this section focuses on localization techniques which rely on both GNSS and non-GNSS systems.
The fact that GNSS and non-GNSS are complementary technologies enables a ubiquitous localization in a wide range of working cases, which may not be feasible by just using one of these technologies. For instance, GNSS localization systems offer an excellent positioning reliability and accuracy if the working conditions are adequate enough (i.e., outdoors). Nevertheless, their performance in harsh environments (i.e., indoor, urban areas) may be compromised due to the attenuation of the signal power or even the loss of signal, the multipath propagation, the presence of interferences such as jamming and spoofing, etc. Conversely, non-GNSS technologies typically provide reliable positioning in indoor and urban scenarios. For example, cellular systems, from second generation (2G) to the emerging fifth generation (5G) are specifically designed for reliable and continuous communications in populated areas, such as indoors and urban, and their signals are able to penetrate buildings and walls, thus making them suitable alternatives for situations where GNSS fails. Similarly, WLAN networks are widely spread indoors nowadays, and their high density and moderate propagation ranges (e.g., a few tens of meters indoors to a few hundred meters outdoors) make them another excellent candidate for offering localization solutions complementary to GNSS. Therefore, the simultaneous use of such technologies by means of hybrid positioning techniques improves the accuracy, fault tolerance, and availability of the localization service both outdoors and indoors.
There are different combinations of hybrid GNSS and non-GNSS, and these can be classified into two main groups: GNSS+INS and GNSS+SoO. Currently, hybrid localization with GNSS and SoO, such as Long Term Evolution (LTE), 5G, UWB, and WLAN, is a hot topic in the localization field.
In the GNSS+INS integration, the short-term stable INS data complements long-term stable GNSS data. These systems can provide more accurate and precise location information than a single system, also yielding information during a possible outage of one system. In GNSS+INS hybrid localization, the inertial information provided by the Micro-Electro-Mechanical Systems (MEMS) provides location relative to a previous location at high rate. Other devices such as cameras, radar, barometers, and many more deliver absolute position information which can also be synchronized with the GNSS signal. Due to this hybridization, the accuracy and ubiquity of the location service is boosted in indoor and urban environments (due to the non-GNSS technologies) while still maintaining the outdoor scenarios (thanks to GNSS technologies). However, INS systems usually require an initial calibration, and they accumulate position error with time.
For GNSS+INS integration, three principle approaches exist: i) loose coupling (combines a GNSS derived position with INS data), ii) tight coupling (integrates GNSS pseudoranges and INS data), and iii) deep coupling (involves INS data in the GNSS signal tracking). Regarding the location privacy of an end user, pure GNSS+INS systems are commendable because only the user equipment aggregates and processes data of both subsystems; no third party is involved.
Similar integration concepts can also be found for the integration of GNSS with terrestrial communication systems. Hybridization of position level is always possible. Many examples for a tighter integration exist as well. For example, GNSS+LTE combines GNSS technologies and cellular-specific technologies, including Observed Time Difference of Arrival (OTDoA), Uplink-Time Difference of Arrival (U-TDoA), and Enhanced-Cell ID (E-CID) to provide a more robust and ubiquitous localization service. Secondly, GNSS+UWB mixes GNSS positioning technologies and UWB technologies, which usually perform Time of Arrival (TOA) techniques. Thirdly, GNSS+WLAN merges GNSS and WLAN technologies, which often employ Received Signal Strength (RSS)-based techniques, to enhance the performance of the localization service.
A sub-group of GNSS+SoO is a heterogeneous IoT system, where GNSS sensors and terrestrial IoT sensors are combined. GNSS technologies suited for IoT receivers are hindered by the requirements of low computational power and low power consumption, which might clash with the computational requirements of GNSS signal processing, thus resulting in a faulty localization service in severe working conditions. In this sense, vendors are developing ultra-low power GNSS modules aimed for IoT mass-market devices, with the objective of providing high accuracy with low-powered sensors.
Many studies have been carried out evaluating these hybrid systems, in particular in autonomous vehicle applications (see J. A. Peral-Rosado et alia in Additional Resources), where security and privacy are mandatory to avoid life-or-death scenarios occasioned by attackers. As hybrid systems use GNSS and non-GNSS technologies, they also suffer from the same security and privacy threats as pure GNSS and pure non-GNSS technologies, and thus the same solutions may be applied (see Location Privacy Challenges and Solutions – Part 1 published in Inside GNSS September/October 2017 as well as later sections of this article). However, as the number of systems in use increases, so does the probability of suffering an attack. In addition, the software required to carry out the hybrid positioning techniques must offer security and privacy, provided by the usual security software. If not, this software becomes a security breach in the hybrid system that can be exploited by attackers.
The threats to location privacy in GNSS+SoO are more obscure and possibly more abundant compared to the case of GNSS+INS because more parties and communication between those parties are involved. The parties involved in a hybrid GNSS positioning system are: the GNSS space segment, the user’s device and the network segment, including the Location Service Provider (LSP), the anonymizer, and the Location Based Service Provider (LBSP), as illustrated in Figure 1 (see inset photo, above right, for all figures). In practice, some of the units shown in Figure 1 can be merged or absent. The main GNSS data regarding the user localization comes from the satellites. Non-GNSS data for localization comes from the LSP. Nonetheless, as compared to a GNSS-based localization, there are several terrestrial entities involved in a non-GNSS localization, as seen in the “Terrestrial positioning system” block from Figure 1.
First, an LSP should offer an entirely software or a hybrid software-hardware solution to the user for his/her positioning. For example, a mobile application for indoor positioning can be downloaded from a certain server. Alternately, a dedicated positioning hardware solution can be installed in a shopping mall (based on WLAN, Bluetooth Low Energy BLE, LED, etc.) and users visiting that particular shopping mall can download the application that uses the dedicated infrastructure. The LBSP is the one offering the services to the user, such as finding an item on a shelf in a supermarket, or finding the cheapest offers for nearby restaurants, etc. The LSP and LBSP are typically distinct entities, and, in order to preserve the users’ privacy, they might interact through the help of a third entity, called an Anonymizer. This is done in order to not send the user’s position in clear from one server to another. The last entity in the chain is, of course, the user mobile device, on which the positioning application is running.
The position can be computed in two ways:
- network-centric approach, when the LSP computes the user’s position and sends it to the user, or
- mobile-centric approach (J. H. Lee), when the LSP only sends some of the information to the user (e.g., training databases, maps, etc.), and the user device computes its final position based on the signals in range and the information received from the network.
Clearly, the second approach more successfully preserves location-privacy than the first one.
As mentioned earlier, hybrid GNSS positioning systems combine several positioning systems, thus they also incorporate the vulnerabilities of these positioning systems. In a hybrid positioning system aggregation, pre- and post-processing of data can almost arbitrarily be divided between LSP and user device as long they are able to share (intermediate) results. These exchanges of information can potentially suffer breaches of location privacy. Analogous to loose and tight GNSS/INS coupling in other hybrid GNSS systems, either positions or other features derived from the signals are used to yield a more robust solution. These features are often ranges or RSS, but any feature unique to a certain location could be used.
The location privacy vulnerabilities of hybrid GNSS systems depend on the data used by the non-GNSS positioning system, i.e., ranges or RSS, and whether the data is fused on the device or on the network by the LSP. Data that is missing at the fusion center must be transmitted to it. Data fusion on the user device reduces communication and is typically the better choice from a privacy point of view.
Non-GNSS Localization
In recent years, we have witnessed the advent of the IoT. Terrestrial IoT can have positioning capabilities either based on the signal strength of powers measured at the receiver side or as intrinsic to a certain IoT standard, such as 5G positioning (A. Dammann et alia; M. Koivisto et alia) or LoRa positioning (B. Ray). WLAN is currently the most widespread non-GNSS localization technology in IoT and it is typically based on RSS measurements. Cellular technologies are also gaining prominence in the IoT positioning field. The legacy cellular systems (2G and 3G) do not explicitly support positioning signals in their standards, but they do have positioning capabilities based, for example, on cell– ID (i.e., positioning of the device inside the coverage areas of the heard transmitters), RSS, time of arrival (TOA), or time difference of arrival (TDOA). In 4G cellular systems or LTE, the Positioning Reference Signals (PRS) have been introduced to support TDOA-based positioning. The 5G emerging cellular concept is based on the assumption of very dense Access Nodes (AN), e.g., even down to 5-10 meters average distances between the AN, and very large bandwidths (e.g., trend to move towards mmWave communications, where the spectrum is still scarcely used or unused). These two features strongly support the capacity of achieving highly accurate positioning and tracking through, for example, combinations of TOA, TDOA, and Angle of Arrival (AOA) solutions. The privacy threats in 5G will likely be related more to the attacks during channel transmission rather than to unsecure or malicious ANs, as the security in 5G has been actively addressed, deeply thought out and optimized.
Another category of emerging communication systems with potential support for positioning is the terrestrial IoT category. For instance, Low Power Wide Area Network (LPWAN) standards such as LoRa, NarrowBand IoT (NB-IoT), enhanced Machine Type Communication (eMTC) or Sigfox, which were incipiently devoted to IoT communications, can also be used for IoT positioning. These technologies are also affected with the security threats of typical cellular and non-GNSS based localization systems.
The main threats of IoT positioning techniques relate to attacks performed on the IoT sensor itself instead of the localization service. In this context, the IoT sensor suffers from similar threats as most non-GNSS localization techniques, which are node-based localization solutions. Finally, in heterogeneous IoT sensor networks, as the hybrid positioning techniques are applied in a control unit and not in the device itself by means of software, the security breach produced by this software is circumvented. It is supposed that the control unit is already protected against attacks which may jeopardize the security and privacy of the sensor or user. More aspects related to hybrid and non-GNSS localization are discussed later.
Passive Positioning Concept
The current literature includes a dual definition of “passive positioning.” The two definitions, used with opposite meanings, are given below:
1. “Passive” from the user’s point of view: the user terminal is passive, meaning that it does not send any positioning information to the network; the terminal only receives signalling or other information relevant to its positioning, similar to a pure GNSS device. Thus, the network does not have any knowledge about the user’s position. The user is the only one responsible for calculating his/her position in a fully mobile-centric mode and the only one who will have that information (see L. Chen et alia and V. Sark et alia).
2. “Passive” in the sense of “uninvolved” or non-participative user, also referred to as “device-free” localization: the user has no idea it is tracked or positioned and the network locates and tracks the user without his/her express authorization, typically in a radar-like approach, by using signal reflections on the users’ devices or body or passive tags. The user terminal can also be seen as “passive” in the sense that the user does not take an active part in the localization process (see N. Pirzada et alia and Z. Zhang et alia).
In this article, we adopt the first definition, as it is the one strictly associated with a privacy-preserving positioning. We also make the distinction here between the LSP, which is typically the network operator or the provider of the actual positioning information, and the LBSP, which is the provider of a certain service that needs the location information. Many times, they are one and the same, but sometimes they can be disjoint, e.g., an LBSP in a shopping mall which advertises the best-value in that shopping mall can take the position information from a separate LSP entity, which might have installed a positioning-specific infrastructure in that particular mall.
Location Privacy in Hybrid- and Non-GNSS-Based Positioning
In contrast to GNSS, the majority of modern communication systems use bidirectional communication and rely on unique identification of their nodes. Thus, the network operator is, in general, able to obtain knowledge of its user’s whereabouts just based upon the proximity to the AN or the transmitter the user is connected to. It already becomes clear that revelation of location information is almost inevitable when using a communication system for positioning purposes. However, to what extent this becomes critical depends primarily on the accuracy of the location information and the context it might be linked to. The following two sections assess the location privacy vulnerabilities of range-free and range-based positioning systems, which translate to hybrid GNSS positioning systems as part of a loosely or tightly coupled, user-centric or network-centric system.
RSS-Based Techniques
Any communication system can also be used deliberately as a positioning system. WLANs are among the most prominent SoO, providing location information as accurate as a consumer-grade GNSS, but are much less protective of privacy. Fingerprinting relies on the concept that signatures of Radio Frequency (RF) signals – typically RSS signatures (i.e., RSSs and corresponding MAC addresses) – are unique at different locations, and that once enough of these signatures are known at sufficient locations, a user’s location can be recognized at a later stage solely by the signature associated with that location. The set of RSS signatures obtained at known locations is known as a radio map or fingerprint database.
The vulnerabilities in terms of privacy of a fingerprinting-based positioning system depend on the type of positioning system/infrastructure. Two typologies are prevalent: a) infrastructure based, or network-centric, and b) terminal based, or mobile-centric. In a network-centric positioning system, the user observes the signal signatures of the network’s ANs and sends them back through the network to the location provider, where the location is retrieved as the position that is associated with the pre-recorded signatures of the radio map that best match the observed signatures. In a mobile-centric system, a copy of the radio map is available on the user’s device and the position is estimated by the device.
Both, network- and mobile-centric positioning systems are prone to breaches of the user’s location privacy due to a communication link that identifies the user device. Let’s consider the scenario of an adversary controlling an untrusted network. The adversary might use the known AN positions to which a user device connects and infer its location roughly based on proximity. The location disclosure type of attack basically depends on the user’s need and perception of his/her location privacy (J. H. Lee et alia) and by the granularity level of the position information disclosure, as discussed in our previous article.
We extend that scenario and assume that the attacker evaluates packets sent by the user at several ANs in range and that the attacker predicts a radio map with a basic pathloss model and knowledge of the AN positions. Now the adversary can use fingerprinting based on the MAC addresses of the ANs that received packets from the user device (MAC addresses are easily obtained by an eavesdropper, as they are transmitted in the clear by most existing WLAN chipsets.). A rank-based fingerprinting (FP) algorithm can be used to match the MAC addresses of the ANs in the range of the user with that of the radio map. The adversary might as well use fingerprinting with RSS signatures to deduce the user’s position even more accurately. In addition to the previous case he would need to evaluate the RSS from the user’s packets at the different AN positions. The symmetry of the channel (ANs’ RSS signature at the user position equates to the user’s RSSs at the ANs’ location) allows one to estimate the user location by matching the observed RSS to a radio map. A test performed in a four-floor university building in Tampere, Finland showed that the accuracy that can be obtained by an eavesdropper for RSS based FP in WLANs is about 8 meters and typically below 10 meters in more than 70-80% of cases, as illustrated in Figure 2.
Figure 2 shows the positioning accuracy (in terms of cumulative distribution of the distance error) that can be obtained by an adversary for the previously mentioned four-floor building. Three different cases are included: i) the adversary has access to the training database (radio map) and to both MAC addresses and RSS measured by the untrusted network from the attacked device (FP method, average accuracy about 8 meters), ii) the adversary has access to the training database and MAC address knowledge (rank based FP method, average accuracy about 17 meters), and iii) no training phase is needed (the radio map is predicted based on a simplified path loss (PL) model) and the adversary uses only MAC address knowledge (PL, rank based FP method, average accuracy about 27 meters). For networks with a low density of untrusted ANs, i.e., a few ANs placed in the building by an adversary, even the last approach would still offer building-level accuracy. If the adversary additionally has an actual radio map (i.e., training database), the average accuracy can decrease to about 17 meters or even 8 meters, depending on the positioning information used (MAC only or MAC+RSS).
In the network-centric setting, the same vulnerabilities exist. Additional risks arise due to the involvement of an LSP, storing and processing the user’s data with his consent, and the transmission of information that is part of the positioning process, for example, the RSS signature measured by the user, or the estimated position that is forwarded by the LSP to the LBSP. Methods to prevent this are addressed later in this article.
While some location-related vulnerabilities can only be exploited if the attacker has access to the network or information about it, others require information about the positioning system. In the worst case, the adversary is an untrusted network operator or LSP who intentionally computes and/or leaks the location data, or who provides unintentional access to information that allows a third party to compute and/or leak the sensitive information.
Assuming a trustworthy LSP/network operator, a mobile-centric positioning system preserves the location privacy better than a network-centric one because of the reduced communication or signaling between the user and the network. The location privacy in a mobile-centric WLAN positioning system can be further protected if the user does not need to associate with an AN and all necessary data for positioning. For example, a fingerprint database or access node position are broadcast while the user device just listens using 802.11’s “monitor mode” (F. Gschwandtner et alia). However, this scenario is limited to special use cases because this mode hinders communications for the user and is usually not enabled by the user.
Further arguments against the mobile-centric approach exist. First, the radio map is a valuable key component for the location service provider, which is therefore reluctant to make it available without obtaining the user’s location information in exchange. Secondly, maintaining multiple copies of the database implies additional costs. Thirdly, the mobile devices might lack sufficient memory and processing power. Thus, network-centric fingerprinting systems are the common case and one question becomes apparent: is the location service provider trustworthy?
If the LSP/network operator cannot be trusted, then an end-to-end encryption is required to preserve location privacy. For a network-centric positioning system, in which the user’s location is estimated by the LSP, end-to-end encryption can be achieved if the required computations are executed on the encrypted data. Homomorphic encryption allows computations on the encrypted data that, once decrypted, equal the result of the same computations performed on the plain data. For example, the Pallier cryptosystem, which provides only additive homomorphism and therefore reduces computational complexity, has been applied to WLAN fingerprinting (H. Li et alia). As the homomorphic property is reduced to additions, more complex operations can be decomposed and precomputed such that the LSP can perform signature matching based on additions only. However, transmitting several precomputed terms increases the communication overheads. Alternative secure two-party computation protocols, such as Additive Sharing, Yao’s Garbled Circuits, might further reduce the computational burden. Their use for RSS-based fingerprinting is currently under investigation.
One might conclude that, in order to achieve reasonable location privacy on the device, an end-to-end encryption is indispensable during communications and at the LSP side. The use of (partially) homomorphic encryption points to a promising direction, however, many practical issues have still to be solved. Given the diversity of pattern matching algorithms used in fingerprinting, the privacy protection scheme must be included in the design of the positioning system.
Timing and Angle-Based Techniques
Typically, timing and angle-based positioning methods require that the user device is communicating with the network. Examples of timing and angle-based positioning solutions widely used in cellular systems are, for example: TOA, TDOA, Round Trip Times (RTT), Time Of Flights (TOF), Angle or Direction of Arrival (AOA/DOA), Differential Direction of Arrival (DDOA), etc. Due to these communications over wireless channels, an untrusted network could get access to the user location information, but due to synchronization, authentication, and signaling requirements in various cellular and non-cellular communication networks, it is much harder for an attacker to build such an untrusted network, compared with the case when only RSS information is used.
An alternative to the situation when the user communicates with the network in order to get his/her position information via timing or angle approaches is the situation when the network broadcasts some signaling messages for all users in range, and such broadcast messages include the location of the network ANs, the starting time of the signaling message, and possibly some additional information, such as the forwarding time between two ANs. This approach has been proposed for the future 802.11az WLAN standard (see Additional Resources) and it is worth mentioning because it can offer a fully privacy-preserving approach, as the user is not sending back any information to the network. The concept is illustrated in Figure 3.
The ANs in a certain area or building are assumed to be synchronized and to belong to a certain LSP. One of the ANs in the network acts as an initiator and starts sending broadcast and forwarding messages in its range. Each AN that receives a forwarding message, re-sends it further with a certain delay (known to the network and broadcast in the broadcasting message). The mobile user receives such broadcast messages from all the ANs in range, and it is able to compute its position via hyperbolic trilateration (V. Sark et alia), as the ANs’ positions are known (transmitted in the broadcast messages). Such a positioning mechanism has recently been studied by E. S. Santiago. It has been found that at least 10 ANs must be in range of the user mobile in order to achieve good location accuracy. A basic open-source simulator for 802.11az-based positioning studies is also available from E. S. Lohan (see Additional Resources).
Methods to Protect Location Privacy
As the discussions so far show, there is an emerging need for protecting user location privacy and various methods and measures have already been studied or adopted. In our previous article, we described several possible methods currently used or proposed to protect location privacy, such as location cloaking, location obfuscation, position sharing, k-anonymity approaches, and mix zones. Table 1 (see inset photo, above right) presents a summary of privacy-preserving or privacy-protecting methods for user wireless localization.
The listed methods have been developed in light of certain attack scenarios and are vulnerable to attacks in which the adversary has further knowledge than originally assumed in the scenario. Here we mention only the base algorithms, to which many extensions exist. In general, the more information and context an adversary can link to the location data, the less effective these privacy-preserving methods will be. According to the protection goal, these context components are typically user location, temporal information, and user identity. Some privacy-preserving methods may need to be combined in order to achieve full user protection.
Conclusions
In comparison to modern GNSS solutions, such as Cloud and Assisted GNSS, where privacy of localization studies have only recently emerged, the location privacy in hybrid- and non-GNSS localization systems is a multi-faceted issue where many solutions have already been studied and published in the research community. These interdisciplinary efforts need to be further consolidated to design privacy-preserving IoT localization technologies and services. There is a clear inherent tradeoff between the granularity of defining the location accuracy by a certain Location Service Provider and the level of location privacy that the user can reach. Herein we have summarized some of the existing solutions for preserving the user’s location privacy. We have also pointed out that the research on location privacy is a worthy endeavor for future positioning systems targeting sub-meter level accuracies.
Acknowledgements
The authors express their warm thanks to the Academy of Finland (Project 303576) for its financial support for this research work.
Additional Resources
[1] Chen L., S. Thombre, K. Jarvinen, E.S. Lohan, A.K. Alen-Savikko, H. Leppäkoski, M.Z.H., Bhuiyan, S. Bu-Pasha, G.N. Ferrara, and H. Honkala, “Robustness, Security and Privacy in Location-Based Services for Future IoT: A Survey,” IEEE Access, 2017
[2] Dammann, A., R. Raulefs, and S. Zhang, S.,“On Prospects of Positioning in 5G,” 2015 IEEE International Conference on Communication Workshop (ICCW), London, pp. 1207-1213, 2015
[3] Gschwandtner, F. and C.K. Schindhelm, “Spontaneous Privacy-Friendly Indoor Positioning using Enhanced WLAN Beacons,” International Conference on Indoor Positioning and Indoor Navigation (IPIN), 2011
[4] Lee, J.H. and R.M. Buehrer, “Security Issues for Position Location,” chapter in Wiley Handbook for Position Location, 2011
[5] Koivisto, M., Costa, M., Werner, J., Heiska, K., Talvitie, J., Leppänen, K., Koivunen, V., and Valkama, M., “Joint Device Positioning and Clock Synchronization in 5G Ultra-Dense Networks,” IEEE Transactions on Wireless Communications, Volume: 16, Issue: 5, pp. 2866-2881, May 2017
[6] Li, H., L. Sun, H. Zhu, X. Lu, and X. Cheng, “Achieving Privacy Preservation in WiFi Fingerprint-Based Localization,” IEEE Conference INFOCOM, 2014
[7] Lohan, E. S., P. Richter, V. Lucas-Sabola, J. Lopez-Salcedo, G. Seco-Granados, H. Leppakoski, and E. Serna Santiago, “Location Privacy Challenges and Solutions – Part 1: GNSS Localization,” Inside GNSS, September/October 2017
[8] Lohan, E.S. et alia, “Open-Source Software and Measurement Data Available at TLTPOS Group, TUT,” accessed June 20, 2017
[9] Peral-Rosado, J.A., R. Estatuet, J.A. Lopez-Salcedo, G. Seco-Granados, G. Chaloupka, L. Ries, and J.A. Garcia Molina, “Evaluation of Hybrid Positioning Scenarios for Autonomous Vehicle Applications,” Proceedings of ION GNSS+, September 2017
[10] Pirzada, N.M.Y., F.S.M.F. Hassan, and M.A. Khan, “Device-Free Localization Technique for Indoor Detection and Tracking of Human Body: A Survey,” Procedia-Social and Behavioral Sciences, Volume: 129, pp. 422–429, 2014
[11] Ray, B., LoRa Localization, Blog entry, June 2016
[12] Sark, V., E. Grass, and J.G. Teran, “Efficient Positioning Method Applicable in Dense Multi User Scenarios,” IEEE 802.11 White Paper, 2016
[13] Serna Santiago, E., Passive Positioning Approaches in the future positioning systems, MSc. Thesis, Tampere University of Technology, May 2017
[14] Zhang, Z., Z. Tian, M. Zhou, Z. Li, Z. Wu, and Y. Jin, “WIPP: Wi-Fi Compass for Indoor Passive Positioning with Decimeter Accuracy,” MDPI Applied Sciences, Volume: 6, p. 108, 2016