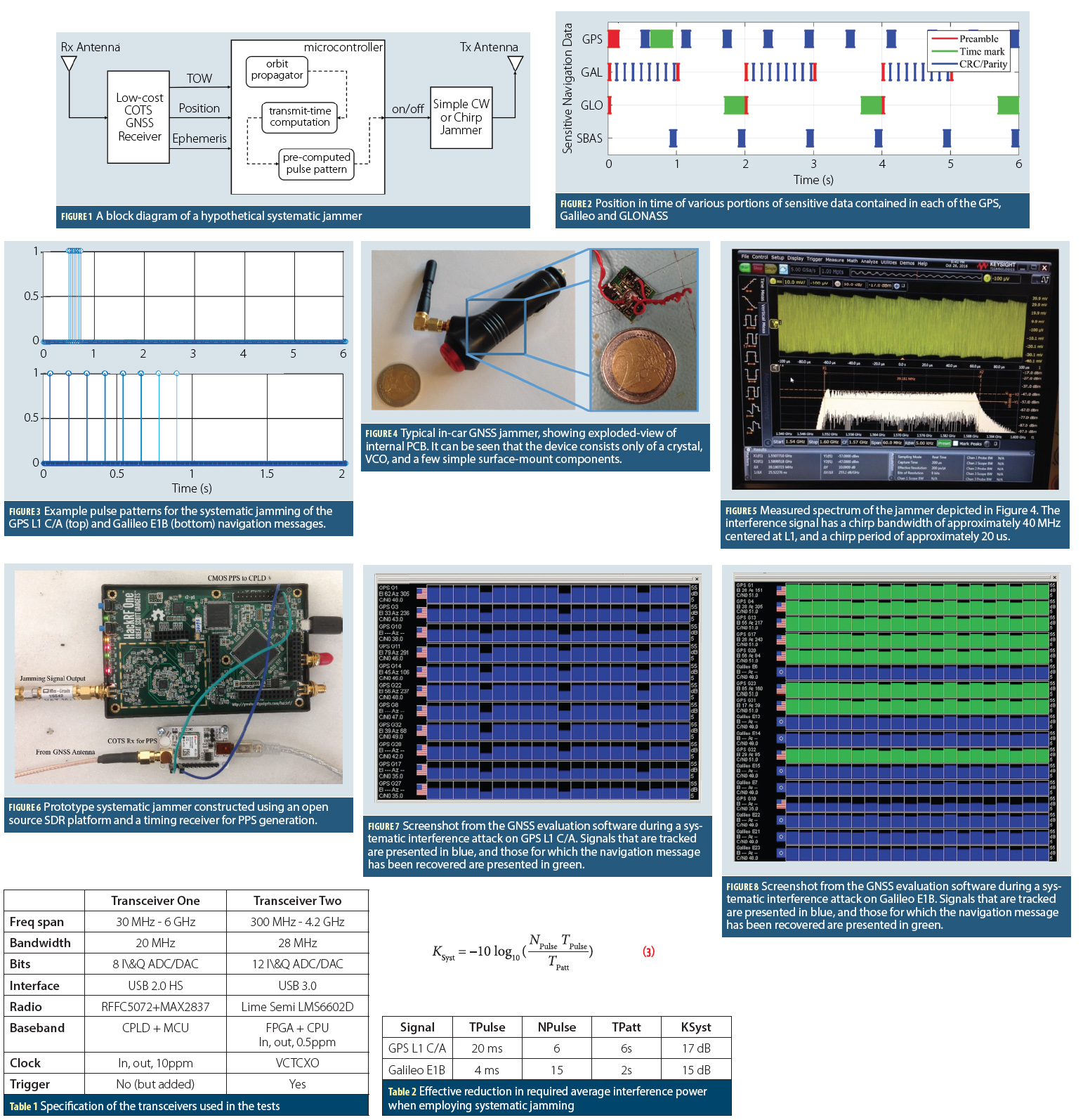
There are many good reasons for getting excited about highly automated vehicles, or HAVs, which is the acronym used by the National Highway Traffic Safety Administration (NHTSA). HAVs can make driving more fuel- and time-efficient. They can significantly reduce traffic congestion and emissions by driving a precise speed, minimizing lane changes, and maintaining an exact distance to neighboring cars. They can also increase accessibility and mobility for disabled and elderly persons.
There are many good reasons for getting excited about highly automated vehicles, or HAVs, which is the acronym used by the National Highway Traffic Safety Administration (NHTSA). HAVs can make driving more fuel- and time-efficient. They can significantly reduce traffic congestion and emissions by driving a precise speed, minimizing lane changes, and maintaining an exact distance to neighboring cars. They can also increase accessibility and mobility for disabled and elderly persons.
Sharing an HAV instead of owning is projected to dramatically reduce a household’s yearly transportation budget, which currently ranges between approximately $8,000 and $11,000 per car. HAVs carry promises not only in improved road mobility, and accessibility, but also in producing architectural and societal changes that can make mass parking spaces and personal car ownership obsolete in urban areas. Above all, HAVs can help improve road safety by preventing car accidents that cause more than 30,000 deaths/year in the United States alone, cost approximately $230 billion/year in medical and work loss costs, and are caused by humans 90% of the time.
Press articles in the 1950s and 1960s predicted that autonomous cars and “electronic highways” would become widely available by 1975. Major milestones in the use of new sensor, computation, and communication technology have recently reenergized the eagerness for HAVs. This first started with the 2005 “DARPA Grand Challenge”, where four different HAVs designed by teams of engineers from industry and academia completed a 132-mile trip across the Mohave desert in less than 7.5 hours with no human intervention. The 2007 DARPA “Urban Challenge” saw six teams autonomously complete a 60-mile course in an urban environment, while following traffic laws. Most teams used a combination of LiDAR, cameras, differential GPS, and computation power that is multiple orders of magnitude higher than what is typically needed for a commercial passenger vehicle. In 2009, Google (now Waymo) began designing and testing “self-driving” cars, which have since accumulated more than three million miles in autonomous mode.
Currently, most car manufacturers have HAV prototype systems and Google, Uber, NuTonomy have HAV pilot testing programs, including fully autonomous systems for public transportation, which, for now, are confined to segregated lanes and geo-fenced areas. Multiple Tier-2 supplier companies have emerged, which specialize in autonomous car technology. In early 2017, 36 companies were registered to test prototype HAV systems on public roads in the state of California.
However, in Figure 1 (for all figures, see inset photo, above right), Gartner’s “2016 Hype Cycle for Emerging Technologies” shows that HAV technology might be at the “peak of inflated expectations”, approaching the “trough of disillusionment”. Hype cycle curves are non-scientific tools that have been empirically verified for multiple example technologies over many years. Two example emerging technologies, commercial unmanned aircraft systems (UAS) and virtual reality, are included in Figure 1 for illustration purposes. The curve’s time scale may differ for each technology. One of many indicators of decreasing expectations on HAVs include a reduction in press coverage and the emergence of first negative news stories, in particular following the May 2016 crash of a Tesla Model S whose autopilot failed to distinguish a white trailer truck from the bright Florida sky. The Model S ran under the trailer causing its roof to be torn off and the operator to lose its life. The car kept going full speed on the side of the road through two fences until it hit a pole and came to a stop.
In parallel, until the end of 2016, Google was providing detailed reports of their self-driving car performance, which were designed to operate in real-world urban environments. These reports contain records of millions of miles driven autonomously, but also acknowledge “disengagements”, i.e., where the operator needed to take over control to avoid collisions. The data shows that HAVs are much more likely to be involved in collisions, even though these collisions are often of lower severity than in conventional human driving [HAVs typically get rear-ended because of their unusual road behavior] (see B. Schoettle, and M. Sivak, “A Preliminary Analysis of Real-World Crashes Involving Self-Driving Vehicles,” Additional Resources). Also, Uber’s autonomous taxis in Pittsburg have a reported rate of one disengagement per mile autonomously driven.
Moreover, the first fielded autonomous systems have revealed new safety threats. In particular, the technology’s functionality, as perceived by the human operator, does not always match the intended operational domain: for example, there have been cases of highway autopilots being used in urban areas and passing red lights without slowing down. In addition, human-machine interaction is at the heart of role confusion (is the operator or the HAV in charge?) of mode confusion (is the HAV in autonomous or manual mode?) and of the operator’s trust in this multimodal system. Misinterpretation may grow even wilder because a given functionality will not achieve the same level of performance across models and manufacturers, and operators may not be aware of the systems’ independently verified safety ratings. And, within the next few years, operators will be expected to anticipate hazardous situations and take over control. Thus, operating an HAV may require more education and different training than driving a car manually.
Current Safety Assessment Efforts
To focus this article, first consider the Society of Automotive Engineer (SAE) International’s classification of driving autonomy levels in Table 1 (see inset photo, above right). Under Levels 0 to 2, the human driver is responsible at all times, either for driving by himself, or for supervising the HAV in autonomous mode and taking control if needed. Under Levels 3 to 5, the system is self-monitoring and the driver is expected to take control, but only if requested by the system. Levels 0-4 provide partial automation under predefined driving modes and circumstances, whereas Level 5 is full autonomy.
The most advanced private car systems are currently Level 2, and pilot programs aim at achieving Level 3, although the mere presence of a kill-switch would imply that the system is actually Level 2. The transition from Level 2 to 3 is a remarkable leap that has significant implications on trust and comfort of human-machine interactions, on legal responsibility allocation between system and driver, and on technical challenges to overcome to guarantee passenger safety.
Over the past four years, the most publicized approaches to demonstrate Level 2 HAV safety have been experimental testing campaigns by Google, Tesla and Uber. Google’s approach to have HAVs drive millions of miles with minimal human intervention has been documented up until 2015. At this time, Google cars have autonomously travelled an impressive three million miles. Tesla’s autopilot is reported to have driven more than 130 million miles – on highways only – before it caused a fatality in May 2016.
In parallel, NHTSA reports about 3,000 billion miles travelled each year on U.S. highways by human drivers, with 30,000 deaths caused by traffic accidents; this corresponds to about one fatality in traffic accidents per 100 million miles driven in the U.S. But, this number accounts for incidents on all roads, in all weather conditions, and for all vehicle ages and types. Thus, a purely experimental, complete proof that HAVs match the level of safety of human driving would take about 400 years at Google’s current testing rate (of approximately 250,000 test miles per year), and would still take many decades if the testing rate increased exponentially. This is assuming that no fatalities occur during that time, that no major HAV upgrade is performed, and that the testing environment is representative of all U.S. roads. Thus, while an experimental proof is conclusive, it is not practical. Other, analytical, methods must be employed to ensure HAV safety.
Research Challenges In HAV Navigation Safety
Multiple technical aspects developed over decades for automated flying could serve as starting points for automated driving systems. Figure 2 shows research areas with overlap between aircraft (in blue) and car (in yellow) applications. Figure 2 is not intended to give a comprehensive list of all aspects of automation, but instead, it shows example technical areas that can be addressed using similar methods in aviation and automotive applications (in the green area). For example:
- performance standards set for software, communication, and electronic equipment are already being compared for aircraft versus cars in the NHTSA report by Q. D. Van Eikema Hommes, Additional Resources.
- the design of aircraft cockpit has been continuously improved over the past few decades, especially for highly-automated Unmanned Air Systems (UAS) with a remote pilot “in-the-box”; few car manufacturers envision futuristic car interiors where humans do not participate in driving, but as long as human-machine interactions are needed, lessons learned in cockpit design to avoid information overload are key.
- while Automatic Dependent Surveillance-Broadcast (ADS-B) will be mandatory on all aircraft by 2020, a petition for proposed rule making has been issued to mandate Vehicle-to-Vehicle (V2V) and Vehicle-to-Infrastructure (V2I) by the same date. (ADS-B is a situational awareness system for collision avoidance, through which aircraft share their positions with Air Traffic Control and with other aircraft.)
- GNSS/INS navigation systems, which are extensively used in safety-critical aircraft navigation, are also being investigated for HAVs.
- overall safety standards also have similarities for aircraft and HAVs, which are discussed again below.
The focus of this article is on navigation safety. In aviation navigation, safety is assessed in terms of integrity (as well as accuracy, continuity, and availability, which are not discussed for brevity). Integrity is a measure of trust in sensor information: integrity risk is the probability of undetected sensor errors causing unacceptably large positioning uncertainty (See RTCA Special Committee 159, “Minimum Aviation System Performance Standards for the Local Area Augmentation System (LAAS), Additional Resources”). This top-level quantifiable performance metric is sensor- and platform-independent, and can thus be used to set certifiable requirements on individual system components to achieve and prove an overall level of safety.
The multiple separate efforts towards achieving Levels 3-to-5 HAVs reveal a compelling lack of coordination towards a common, uniform, quantifiable safety goal. Integrity can be used as an objective performance metric for open, transparent comparison and categorization across manufacturers. It can also provide a governmental regulating agency performance and testing standards for HAV certification, which would help accelerate the development, growth, and maturation of such HAVs, as displayed in Figure 3.
Moreover, the Federal Aviation Administration (FAA) has developed analytical methods to evaluate integrity. This provides the means to:
- quantify safety of existing multi-sensor systems under a variety of operating environments, thereby reducing the need for experimental testing
- allocate safety requirements to individual system components to achieve an overall target level of safety, thereby enabling design for safety
- perform risk prediction, which is a key operational feature to enable hazard avoidance maneuvers
Several methods have been established to predict the integrity risk in GNSS-based aviation applications, which are instrumental in ensuring the safety of pilots and crew. As an example, Figure 4 illustrates a simplified definition of the integrity risk for aircraft landing applications. The aircraft positioning prediction is uncertain because of sensor measurement noise. An alert limit (AL) requirement box is represented around the predicted aircraft position. This AL is set by the certification authority, i.e., by the FAA in this application. Simply put, the risk of the actual aircraft position being outside the AL box is the integrity risk. (In practice, the most challenging part of risk prediction is to account for potentially undetected sensor faults, such as excessive GNSS satellite clock drift.)
Unfortunately, the same methods do not directly apply to HAVs, because ground vehicles operate under sky-obstructed areas where GNSS signals can be altered or blocked by buildings and trees. In general, the HAV environment is much more unpredictable than the aircraft’s, for reasons that include:
- a changing environment: traffic lights, construction, impact of rain on road adherence, sensor masking and occlusions,
- environmental diversity: intersection topography, road conditions, markings on ground, various traffic signs
- road users that may interfere with HAV motion: other cars, trucks, pedestrians, bicyclists, etc.
- comparatively large number of car manufacturers, equipment suppliers, and vehicle models, as well as with shorter model cycles than aircraft, causing wide variations in vehicle age and maintenance levels
- non-uniform vehicle and road regulations at both the state and federal levels in the U.S. coupled with different international standardization processes.
Thus, HAVs require sensors in addition to GNSS, including laser scanners, radars, cameras, and odometers.
The parallel between aircraft and car applications in Figure 4 illustrates the significant challenge that lies ahead when bringing aviation safety standards to HAVs. It took decades of research and considerable resources to bring the alert limit requirement box down to 10 meters above and below the aircraft using the FAA’s GPS augmentation systems (the Wide-Area Augmentation System and the Local Area Augmentation System). For a car to stay in its lane, the alert limit requirement box must be an order of magnitude smaller, and has to maintain this level of safety in a more dynamic and unpredictable environment.
HAV Taxonomy
Creating a path to successful automated navigation requires an overall methodology to prioritize on imminently achievable objectives, and then expand to more challenging missions. First in this HAV taxonomy, a classification using six SAE autonomy levels has been presented in Table 1. This classification is further refined by segmenting a car’s trip into basic driving competencies, and by specifying the conditions under which a given HAV shall achieve these competencies. A similar classification was made in the early days of GPS-based commercial aircraft navigation safety analysis, where distinctions were made between different phases of flight, weather conditions, vehicle equipment, and airport infrastructure capabilities.
For example, in the early 1990’s, 40% of aircraft accidents were occurring during final approach and landing, and 26% during take-off and initial climb, which only represented an average of 4% and 2% of flight time, respectively. The FAA therefore concentrated their efforts on improving safety during these phases of flight. GPS augmentation systems were designed, with varying capabilities depending on airborne equipment and airport infrastructure, to guide the aircraft under the cloud ceiling, or to bring it all the way to touch-down. Similarly, the “first and last mile” are identified as the most challenging parts of HAV operations, whereas highway auto-drive systems have already been developed and implemented. In its 2016 Federal Automated Vehicles Policy, NHTSA identifies 28 HAV behavioral competencies, which are particularly challenging to meet in the first and last miles of a typical trip. These competencies are basic abilities that an HAV must have to complete nominal driving tasks; they include, for example, lane keeping, obeying traffic laws, and responding to other road users.
To better describe an HAV’s ability, the Federal Automated Vehicles Policy further specifies that basic driving competencies should be available under an HAV’s predefined Operational Design Domain (ODD), described by its geographical location, road type and condition, weather and lighting condition, vehicle speed, etc. The ODD captures the circumstances under which an HAV is supposed to operate safely.
Such classification is key to safety analysis. It can allow HAVs at different stages of their development to be simultaneously fielded, and for them to evolve by expanding their ODDs. The classification can also help in identifying geographical areas where improved road infrastructure is needed for automated operation, similar to airports requiring equipment for instrument navigation to deal with higher traffic density.
Furthermore, standards for electronic equipment, measured by Automotive Safety Integrity Levels, have been issued and compared with the aviation’s Design Assurance Levels (DAL). And, overall system safety levels have been codified, which in aviation account for both the severity and probability of occurrence of an incident, and in automotive applications account, in addition, for “controllability”, which is a measure of how likely an average driver is to maneuver out of a given imminent danger.
All of the above elements: (a) HAV autonomy level, (b) basic driving competency, (c) operation design domain, (d) vehicle electronic equipment, and (e) overall safety risk requirement must be specified to carry out a formal HAV safety analysis. Still missing from the HAV documents are clear guidelines, or example methods, on how to implement these safety requirements.
A Path Towards HAV Navigation Safety
When quantifying the safety of HAV navigation systems, such as in the example displayed in Figure 5, every component of the system including raw sensors, estimator and integrity monitor, and safety predictor, can potentially introduce risk. Unlike aircraft, HAVs require multiple and varied sensors to compensate for GPS signal blockages caused by buildings and trees. These sensor types must be integrated, and new methods to evaluate the integrity of multi-sensor systems must be developed. Furthermore, HAVs must have the ability to continuously predict integrity in a dynamic HAV environment.
In general, research on analytical evaluation of HAV navigation safety is sparse. For example, J. Lee et alia, Additional Resources use the concept of a “safe driving envelope,” but the approach focuses mostly on collision avoidance. The paper by O. Le Marchand, et alia, evaluates ground vehicle navigation, but shows an “approximate radial-error” of tens of meters, far exceeding the necessary sub-meter alert limit. A multi-sensor augmented-GPS/IMU system is used in the paper by R. Toledo-Moreo, et alia with “horizontal trust levels” of 7 meters to 10 meters, still an order-of-magnitude higher than the required HAV alert limit.
Multi-sensor integrity is addressed by M. Brenner, Additional Resources, but for a sensor combination specific to aviation and insufficient for terrestrial mobile robots. Other approaches to multi-sensor integration show promise, but do not provide rigorous proof of integrity. In fact, most publications use pose estimation error covariance as a measure of performance, which is understood as not being sufficient, but is the only metric currently available. Most critically, the metric does not account for fault modes introduced by feature extraction and data association, two algorithms commonly used in mobile robot localization (and discussed again below).
Unlike GPS, which gives absolute position fixes, IMUs, LiDAR, radar, and cameras provide relative displacements with respect to a previous time-step, or with respect to a map. Thus, measurement time-filtering is required, which makes integrity risk evaluation more challenging since past-time sensor errors and undetected faults can now impact current-time safety.
Example LiDAR Navigation Safety Evaluation
While safety quantification for GNSS and GNSS/INS has been rigorously performed for aviation applications, and is being researched for HAVs, navigation safety for LiDAR, radar, camera, and multi-sensor navigation is a widely unexplored research area. To provide a specific example on the research work that lies ahead, we have started developing safety risk evaluation methods for LiDARs. We selected LiDARs because of their prevalence in HAVs, of their market availability, and because of our prior experience. However, the techniques we are developing are general enough that radar, cameras, or any future sensor that returns range data can be substituted.
Raw range data must be processed before it can be used for navigation. One technique, visual odometry, establishes correlations between successive scans to estimate sensor changes in pose (i.e., position and orientation). These processes are highly computationally intensive, and have the same problems as other dead-reckoning techniques, such as wheel odometry over time. Thus, they can become inaccurate or cumbersome for HAVs moving over multiple time epochs. Although proprietary information regarding the use of visual odometry by HAV manufacturers is unavailable, the research literature suggests that it is only used for short time scale operations. A second class of algorithms provides sensor localization by extracting static features from the raw sensor data and associating those features to a map. This is typically done in two steps, as illustrated in Figure 6: feature extraction (FE) and data association (DA). The resulting information can then be iteratively processed using sequential estimators (e.g., Extended Kalman filter or EKF), which has been readily used in many practical applications.
There are several problems that the FE and DA algorithms are addressing. First, landmarks in the environment are unidentified, and their observations are not tagged in a manner similar to a GNSS satellite signal’s Pseudo Random Noise (PRN) number. Thus, the feature extraction algorithm must isolate the few most consistently identifiable, viewpoint-invariant landmarks in the raw sensor data. These features must be identifiable over repeated observations and distinguishable from one landmark to another. Features that are difficult to distinguish from each other can be found easily, but the possibility that the association is incorrect will greatly negatively impact the integrity risk.
Second, range data based on extracted features must match those features with those from a feature database or map. Data association algorithms accomplish this; however, incorrect associations commonly occur. These can lead to large navigation errors, as illustrated in Figure 6, thereby representing a threat to navigation integrity.
FE and DA can be challenging in the presence of sensor uncertainty. This is why many sophisticated algorithms have been devised. But, how can we prove whether these FE and DA methods are safe for life-critical HAV navigation applications, and under what circumstances? These research questions are currently unanswered. The most relevant publications on DA risk are found in literature on multi-target tracking. For example, in the paper Y. Bar-Shalom and T. E. Fortmann, an innovation-based nearest-neighbor DA criterion is introduced, which serves as basis in many practical implementations. The article by Y. Bar-Shalom, et alia, “The Probabilistic Data Association Filter,” provides a detailed derivation of the probability of correct association given measurements. However, this Bayesian approach is not well suited for safety-critical applications due to the lack of risk prediction capability, and to the problem of bounding the a-posteriori probability of association (a similar issue is encountered in the paper by F.C. Chan, et alia. Another insightful approach is followed in the paper by J. Areta, et alia). However, it makes approximations that do not necessarily upper-bound risks, hence do not guarantee safe operation, and it presents exact solutions that can only be evaluated using computationally expensive numerical methods, not adequate for real-time navigation. Also, the risk of FE is not addressed.
In response, we have been developing a new, computationally-efficient integrity risk prediction method to ensure safety of localization using LiDAR-based FE and DA. We have derived a multiple-hypothesis innovation-based DA method that provides the means to predict the probability of incorrect associations considering all potential landmark permutations. (For more details on these methods, see the following four papers in Additional Resources, Nos. 31, 49, 50 and 51.) We also determined a probabilistic lower bound on the minimum feature separation, which is guaranteed at FE, with pre-defined integrity risk allocation. The separation bound can be incorporated in an overall integrity risk equation. This new method was analyzed and tested to quantify the impact of incorrect associations on integrity risk. It showed that the positioning error covariance can be a misleading safety performance metric since cases were found where the contributions of incorrect associations to integrity risk far surpassed that of nominal errors accounted for in the positioning error covariance. In addition, the following key safety-tradeoff was illustrated: the more measurements are extracted, the lower the integrity risk contribution is under the correct association hypothesis, but the higher the other integrity risk contributions become because the risk of incorrect associations increases in the presence of cluttered, poorly-distinguishable landmarks. Finally, being surrounded by many landmarks increases the probability of continuous, uninterrupted navigation. The next step of this research aims at dealing with unmapped and non-static obstacles, and at quantifying the continuity risk of FE and DA.
Conclusion
Looking at the emergence of future HAV technology with the prior experience of aircraft navigation safety provides the means to scale up the challenges that lie ahead in the development of fully autonomous (Level 4 and 5) driverless cars. Many parallels can already be drawn between aviation safety requirements and early HAV standards and regulations. Still, the methods to fulfill these standards and regulations have to be established. If analytical methods are pursued, the following tasks need to be accomplished: (1) establish high-integrity raw sensor measurement error and fault models for non-GPS sensors; (2) develop analytical methods to quantify the safety risk of feature extraction and data association algorithms required in LiDAR, radar, and other pre-processing steps in camera-based localization; (3) design multi-sensor pose estimators and integrity monitors to evaluate the impact of undetected sensor faults on safety risk; and (4) derive, analyze, and experimentally implement integrity risk prediction in dynamic environments.
If these challenges are overcome, one will be able to quantify and prove the performance of an HAV’s navigation system — an essential part of safety. Proving navigation system integrity will also help give humans more confidence to trust HAVs, thus further developing the symbiotic relationship between humans and co-robots. Finally, as HAV technology progresses from driver’s aids such as active brake assist to full autonomous driving, this research is relevant now and will remain essential throughout the evolution of HAV technology.
Additional Resources
[1] Abuhashim, T.S., M.F. AbdelHafez, and M.-A. AlJarrah. Building a robust integrity monitoring algorithm for a low cost gps-aided-ins system. International Journal of Control, Automation, and Systems, 8(5):11081122, 2010.
[2] Ackerman , E., “Self-Driving Cars Were Just Around the Corner—in 1960”, IEEE Spectrum, September 2016
[3] Ackerman, E., “After Mastering Singapore’s Streets, NuTonomy’s Robo-taxis Are Poised to Take on New Cities,” IEEE Spectrum, 2016.
[4] Areta, J., Y. Bar-Shalom, and R. Rothrock, “Misassociation Probability in M2TA and T2TA,” J. of Advances in Information Fusion, Vol. 2, No. 2, 2007, pp. 113-127.
[5] Bailey, T., Mobile Robot Localization and Mapping in Extensive Outdoor Environments. PhD thesis, The University of Sydney, 2002.
[6] Bailey, T., and J. Nieto. Scan-slam: Recursive mapping and localization with arbitrary-shaped landmarks. In Workshop at the Institute of Electrical and Electronics Engineers Robotics Science and Systems (IEEE RSS), 2008.
[7] Bakhache, B., A Sequential RAIM Based on the Civil Aviation Requirements. In Proceedings of the 12th International Technical Meeting of the Satellite Division of the Institute of Navigation (ION GPS 1999), pages 1201–1210, 1999.
[8] Basnayake, C., M. Joerger, and J. Aulde, “Safety-Critical Positioning for Automotive Applications”, Inside GNSS Webinar, 2016.
[9] Bar-Shalom, Y., F. Daum, and J. Huang, “The Probabilistic Data Association Filter,” IEEE Control Systems Magazine, 2009, pp. 82-100.
[10] Bar-Shalom, Y., and T. E. Fortmann. Mathematics in Science and Engineering, chapter Tracking and Data Association. Academic Press, 1988.
[11] Bengtsson, O., and A.J. Baerveldt, “Robot localization based on scan-matching-estimating the covariance matrix for the IDC algorithm,” Robotics and Autonomous Systems, Vol. 44, 2003, pp. 29–40.
[12] Bonanni, R., “WAAS – LPV Airport and Aeronautical Surveys”, ANM Airports Conference, 2006.
[13] Bhuiyan, J., “Uber’s autonomous cars drove 20,354 miles and had to be taken over at every mile, according to documents,” available online here, 2016
[14] Blom, H.A.P., and Y. Bar-Shalom. The interacting multiple model algorithm for systems with markovian switching coefficients. IEEE Transactions on Automatic Control, 33(8):780783, 1988.
[15] Brenner, M., Integrated GPS/Inertial Fault Detection Availability. NAVIGATION, Journal of The Institute of Navigation, 43(2):111–130, 1996.
[16] Chan, F.C., M. Joerger, S. Khanafseh, and B. Pervan, “Bayesian Fault-Tolerant Position Estimator and Integrity Risk Bound for GNSS Navigation,” Journal of Navigation of the RIN, available on CJO2014, doi:10.1017/S0373463314000241, 2014.
[17] Chow, E., and A. Willsky. Analytical redundancy and the design of robust failure detection systems. IEEE Transactions on Automatic Control, 29(7):603614, 1984.
[18] Choukroun, D., and J. Speyer. Mode estimation via conditionally linear filtering: Application to gyro failure monitoring. AIAA Journal of Guidance, Control, and Dynamics, 65(2):632644, 2012.
[19] Clot, A., C. Macabiau, I. Nikiforov, and B. Roturier. Sequential RAIM Designed to Detect Combined Step Ramp Pseudo-Range Error. In Proceedings of the 19th International Technical Meeting of the Satellite Division of the Institute of Navigation (ION GNSS 2006), page 26212633, 2006.
[20] ooper, A.J., A Comparison of Data Association Techniques for Simultaneous Localization and Mapping. PhD thesis, Massachusetts Institute of Technology, 2005.
[21] DARPA, “The Six Finishers of the DARPA Urban Challenge,” available online here, 2007.
[22] Defense Advanced Research Projects Agency (DARPA), “Robots conquer DARPA Grand Challenge,” Press Release, U.S. Department of Defense (DoD), 2005.
[23] Department of Transportation (DOT) National Highway Traffic Safety Administration (NHTSA) “Federal Automated Vehicles Policy: Accelerating the Next Revolution In Roadway Safety,” 2016
[24] Diesel, J., and S. Luu. GPS/IRS AIME: Calculation of Thresholds and Protection Radius Using Chi-Square Methods. In Proceedings of the 8th International Technical Meeting of the Satellite Division of The Institute of Navigation (ION GPS 1995), page 19591964, 1995.
[25] Dionne, D., Y. Oshman, and D. Shinar. Novel adaptive generalized likelihood ratio detector with application to maneuvering target tracking. AIAA Journal of Guidance, Control, and Dynamics, 29(2):465474, 2006.
[26] Diosi, A., and L. Kleeman, “Laser scan matching in polar coordinates with application to SLAM,” Proc. IEEE/RSJ IROS, 2005.
[27] Dissanayake, G., P. Newman, S. Clark, H. Durrant-Whyte, and M. Csorba. A Solution to the Simultaneous Localization and Map Building (SLAM) Problem. IEEE Transactions on Robotics Automation, 17(3):229–241, 2001.
[28] Dougherty, M., “Caltrans Leadership in Automated Vehicle Research,” Automated Vehicles Symposium 2017 (AVS2017), San Francisco, CA, 2017.
[29] Dragalin, V.P., A.G. Tartakovsky, and V.V. Veeravalli. The interacting multiple model algorithm for systems with markovian switching coefficients. IEEE Transactions on Information Theory, 45(7):24482461, 1999.
[30] Dragalin, V.P., A.G. Tartakovsky, and V.V. Veeravalli. Multihypothesis sequential probability ratio tests. ii. accurate asymptotic expansions for the expected sample size. IEEE Transactions on Information Theory, 46(4):13661383, July 2000.
[31] Duenas-Arana, G., M. Joerger, and M. Spenko, “Minimizing Integrity Risk via Landmark Selection in Mobile Robot Localization,” submitted to IEEE TRA, 2017.
[32] FAA, “System Design and Analysis,” Advisory Circular AC 25.1309-1A, 1988.
[33] FAA, “System Safety Design and Analysis for Part 23 Airplanes”, Advisory Circular AC 23.1309-1E, 2011.
[34] fars.NHTSA.dot.gov, “Fatality analysis reporting system,” Technical report, NHTSA, 2014.
[35] Federal Aviation Administration (FAA), “Automatic Dependent Surveillance-Broadcast Operations,” Advisory Circular AC No: 90-114A, DoT FAA, 2016.
[36] Federal Highway Administration (FHWA), “Vehicle Positioning Trade Study for ITS Applications”, FHWAJPO-12-064, 2012.
[37] Fenton, R. E., and K. W. Olson “The electronic highway” IEEE Spectrum, 1969.
[38] Forsberg, “NovAtel Establishes Advanced Research Partnership with Illinois Institute of Technology and the University of Arizona,” press release, 2016, available here.
[39] Gartner’s “2016 Hype Cycle for Emerging Technologies” available online here.
[40] Gertler, J., A survey of model based failure detection and isolation in complex plants. IEEE Control Systems Magazine, 8(6):3–11, 1988.
[41] Gitlin, J., “Prepare for the part-time self-driving car,” online at arstechnica.com, 2014.
[42] Google, “Google self-driving car testing report on disengagements of autonomous mode”, available online here, December 2015.
[43] Greenblatt, J. B., and S. Saxena, “Autonomous taxis could greatly reduce greenhouse-gas emissions of us light-duty vehicles,” Nature Climate Change, 5:860–863, 2015.
[44] Greiling Keane, A., “U.S. highway deaths decline for a fifth year, longest streak since 1899,” Bloomberg, Published December 08, 2011.
[45] Halsey III, A., and M. Laris, “Blind man sets out alone in Google’s driverless car,” The Washington Post, 2016.
[46] Hewitson, S., and J. Wang. Extended Receiver Autonomous Integrity Monitoring (eRAIM) for GNSS/INS Integration. Journal of Surveying Engineering, 136(1):13–22, 2010.
[47] Hype cycle curves available online here.
[48] International Organization for Standardization (ISO), “Road vehicles – Functional safety”, ISO 26262, 2011.
[49] Joerger, M., “Carrier Phase GPS Augmentation Using Laser Scanners and Using Low Earth Orbiting Satellites,” Ph.D. Dissertation, Illinois Institute of Technology, 2009.
[50] Joerger, M., M. Jamoom, M. Spenko, and B. Pervan, “Integrity of Laser-Based Feature Extraction and Data Association,” Proc. IEEE/ION PLANS 2016, Savannah, GA, 2016, pp. 557-571.
[51] Joerger, M., B. Pervan, “Continuity Risk of Feature Extraction for Laser-Based Navigation,” Proceedings of the 2017 International Technical Meeting of The Institute of Navigation, Monterey, California, January 2017, pp. 839-855.
[52] Joerger, M., and B. Pervan, “Quantifying Safety for Laser-based Navigation,” submitted to IEEE TAES, 2017.
[53] Kalra, N., and S. Paddock, “Driving to safety: How many miles of driving would it take to demonstrate autonomous vehicle reliability?” Technical Report RR-1478-RC, Rand Corporation, 2016.
[54] Kavanaugh-Brown, J., “Where the Research Meets the Road: Automated Highway Passes the Test ”, Government Technology, 1997. available here.
[55] Kelly, R., and J. Davis, “Required Navigation Performance (RNP) for Precision Approach and Landing with GNSS Application,” NAVIGATION, 1994.
[56] Lee, Y.C., “Analysis of Range and Position Comparison Methods as a Means to Provide GPS Integrity in the User Receiver,” Proc. of the 42nd Annual Meeting of The Institute of Navigation, Seattle, WA, 1986.
[57] Lee, J., B. Kim, J. Seo, K. Yi, J. Yoon, and B. Ko. Automated driving control in safe driving envelope based on probabilistic prediction of surrounding vehicle behaviors. Society of Automotive Engineers International Journal of Passenger Cars – Electronic and Electrical Systems, 8(1):207–218, 2015.
[58] Le Marchand, O., Philippe Bonnifait, Javier Ibaez-Guzmn, and David Btaille. Vehicle Localization Integrity Based on Trajectory Monitoring. In IEEE/RSJ International Conference on Intelligent Robots and Systems, pages 3453–3458, 2009.
[59] Leonard, J., and H. Durrant-Whyte. Directed Sonar Sensing for Mobile Robot Navigation. Kluwer Academic Publishers, 1992.
[60] Li, Y., and Olson E.B. A general purpose feature extractor for light detection and ranging data. Sensors, 10(11), 2010.
[61] Lorden, G., Procedures for reacting to a change in distribution. The Annals of Mathematical Statistics, 42(6):18971908, 1971.
[62] Lu, F., and E. Milios, “Globally Consistent Range Scan Alignment for Environment Mapping,” Autonomous Robots 4, 1997, pp. 333-349.
[63] Madhavan, R., H. Durrant-Whyte, and G. Dissanayake. Natural landmark-based autonomous navigation using curvature scale space. In Proceedings of the Institute of Electrical and Electronics Engineers International Conference on Robotics and Automation (IEEE ICRA), 2002.
[64] Malladi, D. P., and J. L. Speyer. A generalized shiryayev sequential probability ratio test for change detection and isolation. IEEE Transactions on Automatic Control, 44(8):1522–1534, 1999.
[65] Maksarov, D., and H. Durrant-Whyte. Mobile Vehicle Navigation in Unknown environments: a Multiple Hypothesis Approach. In IEEE Proceedings on Control Theory Applications, volume 142, pages 385–400, 1995.
[66] National Transport Safety Board (NSTB), “Preliminary Report, Highway HWY16FH018,” Accident Report ID: HWY16FH018, 2016. available online here.
[67] Neville, K., and K. Williams, “Integrating Remotely Piloted Aircraft Systems into the National Airspace System,” Remotely Piloted Aircraft Systems: A Human Systems Integration Perspective, Wiley, 2017.
[68] Nguyen, V., A. Martinelli, N. Tomatis, and R. Siegwart. A comparison of line extraction algorithms using 2d laser rangefinder for indoor mobile robotics. In Proceedings of the Institute of Electrical and Electronics Engineers/Robotics Society of Japan International Conference on Intelligent Robots and Systems (IEEE/RSJ IROS), 2005.
[69] NHTSA “National motor vehicle crash causation survey: Report to congress,” Technical Report DOT HS 811 059, U.S. Department of Transportation, 2008.
[70] NHTSA, “Federal Motor Vehicle Safety Standards; V2V Communications, Notice of Proposed Rulemaking (NPRM),” DoT NHTSA, 49 CFR Part 571, RIN 2127-AL55, 2016, available online here.
[71] NHTSA “Assessment of Safety Standards for Automotive Electronic Control Systems”, DOT HS 812 285, 2016.
[72] Nikiforov, I., New Optimal Approach to Global Positioning System/Differential Global Positioning System Integrity Monitoring. AIAA Journal of Guidance, Control, and Dynamics, page 10231033, 1996.
[73] Nunez, P., R. Vazquez-Martin, J.C. del Toro, and A. Bandera. Feature extraction from laser scan data based on curvature estimation for mobile robotics. In Proceedings of the Institute of Electrical and Electronics Engineers International Conference on Robotics and Automation (IEEE ICRA), 2006.
[74] Othman, N.A., and H. Ahmad. The analysis of covariance matrix for kalman filter based slam with intermittent measurement. In Proceedings of the 2013 International Conference on Systems, Control and Informatics, 2013.
[75] Page, E.S., Continuous inspection schemes. Biometrika, 41(1-2):100–115, 1954.
[76] Parkinson, B.W., and P. Axelrad, “Autonomous GPS Integrity Monitoring Using the Pseudorange Residual,” NAVIGATION, Vol. 35, No. 2, 1988.
[77] Pfister, S.T., K.L. Kriechbaum, S.I. Roumeliotis, and J.W. Burdick. Weighted range sensor matching algorithms for mobile robot displacement estimation. In Proceedings of the Institute of Electrical and Electronics Engineers International Conference on Robotics and Automation (IEEE ICRA), 2002.
[78] Pfister, S.T., S.I. Roumeliotis, and J.W. Burdick. Weighted line fitting algorithms for mobile robot map building and efficient data representation robotics and automation. In Proceedings of the Institute of Electrical and Electronics Engineers International Conference on Robotics and Automation (IEEE ICRA), 2003.
[79] Radio Technical Commission for Aeronautics (RTCA), “Minimum Operating Performance Standards (MOPS) for Universal Access Transceiver (UAT) Automatic Dependent Surveillance – Broadcast (ADS-B),” RTCA, Washington DC, 2009.
[80] RTCA Special Committee 159, “Minimum Aviation System Performance Standards for the Local Area Augmentation System (LAAS),” RTCA/DO-245, 2004.
[81] RTCA Special Committee 159, “Minimum Operational Performance Standards for Global Positioning System/Wide Area Augmentation System Airborne Equipment,” RTCA/DO-229C, 2001.
[82] Reimer, B., “Revisiting the Topic – The Future is Autonomous Driving – But Are “We” on a Near Term Collision Course?” Automated Vehicle Symposium 2017, (AVS2017), San Francisco, CA, 2017.
[83] Röfer, T., “Using Histogram Correlation to Create Consistent Laser Scan Maps,” Proc. IEEE IROS-2002, Lausanne, Switzerland, 2002, pp. 625-630.
[84] Rogowsky, M., “The Truth About Tesla’s Autopilot Is We Don’t Yet Know How Safe It Is”, Forbes, 2016.
[85] SAE International, “Surface Vehicle Recommended Practice: Taxonomy and Definitions for Terms Related to Driving Automation Systems for On-Road Motor Vehicles,” SAE Standard J3016, 2016.
[86] Schoettle, B., and M. Sivak, “A Preliminary Analysis of Real-World Crashes Involving Self-Driving Vehicles,” Report No. UMTRI-2015-34, October 2015.
[87] Sobel, M., and A.Wald. A sequential decision procedure for choosing one of three hypotheses concerning the unknown mean of a normal distribution. The Annals of Mathematical Statistics, 20(4):502522, 1949.
[88] Soloviev, A., D. Bates, and F. van Graas. Tight Coupling of Laser Scanner and Inertial Measurements for a Fully Autonomous Relative Navigation Solution. NAVIGATION, Journal of The Institute of Navigation, 54(3):189 – 205, 2007.
[89] Soloviev, A., Multi-Sensor Fusion for Navigation of Autonomous Vehicles. In Proceedings of the 26th International Technical Meeting of The Satellite Division of the Institute of Navigation (ION GNSS+ 2013), pages 3615 – 3632, 2013.
[90] Soloviev, A., C. Yang, M. Veth, and C. Taylor. Assured Vision Aided Inertial Localization. In Proceedings of the 27th International Technical Meeting of The Satellite Division of the Institute of Navigation (ION GNSS+ 2014), pages 2160 – 2173, 2014.
[91] Sukkarieh, S., E.M. Nebot, and H.F. Durrant-Whyte. A high integrity imu/gps navigation loop for autonomous land vehicle applications. IEEE Transactions on Robotics and Automation, 51(3):572578, 1999.
[92] Toledo-Moreo, R., M. A. Zamora-Izquierdo, B. beda Miarro, and A. F. Gmez-Skarmeta. High-Integrity IMMEKF-Based Road Vehicle Navigation With Low-Cost GPS/SBAS/INS. IEEE Transactions on Aerospace and Electronic Systems, 8(3):491–511, 2007.
[93] Tena Ruiz, I., Y. Petillot, D.M. Lane, and C. Salson. Feature extraction and data association for AUV concurrent mapping and localization. In Proceedings of the Institute of Electrical and Electronics Engineers International Conference on Robotics and Automation (IEEE ICRA), 2001.
[94] Thrun, S., W. Burgard, and D. Fox. A probabilistic approach to concurrent mapping and localization for mobile robots. Machine Learning and Autonomous Robots, 31(5):1–25, 1998.
[95] Thrun, S., W. Burgard, and D. Fox. A real-time algorithm for mobile robot mapping with applications to multi-robot and 3d mapping. In Proceedings of the Institute of Electrical and Electronics Engineers International Conference on Robotics and Automation (IEEE ICRA), 2000.
[96] Thrun, S., “Robotic Mapping: A Survey,” Exploring Artificial Intelligence in the New Millenium. Morgan Kaufmann Publishers Inc., 2003.
[97] Thrun, S., “National Highway Traffic Safety Administration (NHTSA),” keynote presentation, ION GNSS 2007, Fort Worth, TX, 2007.
[98] Van Eikema Hommes, Q. D., “Assessment of safety standards for automotive electronic control systems,” NHTSA Report No. DOT HS 812 285, Washington, DC, 2016.
[99] Waymo, “We’ve reached 3 million miles of selfdriving on public roads! That’s 1 million miles in just 7 months,” available online here, 2017.
[100] White, N. A., P.S. Maybeck, and S.L. DeVilbiss. Detection of interference/jamming and spoofing in a dgps-aided inertial system. IEEE Transactions on Aerospace and Electronic Systems, 34(4):12081217, 1998.
[101] Wikipedia , “Automotive Safety Integrity Level,” 2017. available here.
[102] Williams, S.B., G. Dissanayake, and H. Durrant-Whyte. An efficient approach to the simultaneous localization and mapping problem. In Proceedings of the Institute of Electrical and Electronics Engineers International Conference on Robotics and Automation (IEEE ICRA), 2002.
[103] Willsky, A. S., A Survey of Design Methods for Failure Detection in Dynamic Systems. Automatica, 12:601–611, 1976.
[104] Working Group C ARAIM Technical Subgroup, “Milestone 3 Report,” Technical report, EU-US Cooperation on Satellite Navigation, 2015.
[105] Yoshida, J., “Another Tesla Crash, What It Teaches Us,” EE Times, 2016.