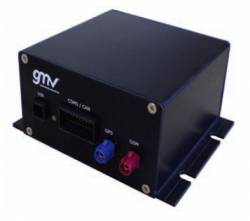
For at least the past two decades, managing traffic on Europe’s road networks has been a growing concern for European policy makers and citizens alike. While demand for transport has consistently increased over the years, Europe’s road network capacity has failed to keep pace, leading to increasing levels of congestion and pollution.
For at least the past two decades, managing traffic on Europe’s road networks has been a growing concern for European policy makers and citizens alike. While demand for transport has consistently increased over the years, Europe’s road network capacity has failed to keep pace, leading to increasing levels of congestion and pollution.
And, although the current economic crisis may have led to a decrease in the volume of goods and passengers transported, future projections all point to a significant increase in transport demand. A modest rebound of the European economy will account for some of this increased demand, but the most significant growth will be fueled by the rapid economic development currently being observed in China, India, and other developing countries.
When considering possible solutions, European governments — gripped by sluggish growth, high levels of public debt, and an aging society — have faced a dilemma. Given that road transport is a vital economic activity that employs 14 million people and accounts for 11 percent of the European Union’s (EU’s) gross domestic product, according to the European Research Transport Advisory Council, policymakers are understandably reluctant to levy additional taxation or other measures that could lead to a slowdown in the sector.
At the same time, things clearly cannot continue under a “business as usual” scenario. From an environmental point of view, the increased transport demand will mean that bottlenecks on Europe’s roads are likely become more and more frequent, leading to higher levels of pollution and noise. This particularly a factor in the region’s cities, which currently account for more than 40 percent of carbon dioxide emissions and more than 70 percent of other emissions, according to data cited by the European Commission (EC) Action Plan on Urban Mobility.
From a financial perspective, the strain on public purses resulting from an aging population means that governments are unlikely to be able to provide the necessary funds for developing new infrastructure, as they are already struggling to preserve existing networks.
Against this backdrop, the idea of road charging as a means of financing roads and managing traffic volumes by making users pay per kilometer has, in recent years, been accepted amongst Europe’s policymakers at the national as well as European level.
This article describes a European research project that investigated the technical and operational aspects of GNSS-based road user charging (RUC). Our discussion will include the results of extensive field trials using an in-vehicle, GNSS-based positioning and data-logging system that took advantage of the increased performances provided by the European GNSS in different configurations and also in combination with other technologies.
The Political Context
At the European level, and in addition to the Eurovignette Directive for taxation of heavy vehicles, the EC’s latest proposals on transport have made clear reference to road charging, which is often coined as “smart pricing” or, in other words, the need for prices to reflect real costs.
This has been coupled with various existing and proposed RUC schemes at the national level. A few examples include:
- charges levied on trucks greater than 3.5 tons traveling across 2,000 kilometers of the Austrian network using a system in operation since 2004 based on Dedicated Short-Range Communications (DSRC) technology
- a German GNSS scheme for trucks above 12 tons on 12,000 kilometers of roads in operation since 2005
- the latest Slovak addition, where authorities in 2010 introduced a GNSS-based charging scheme for heavy goods vehicles (HGVs) greater than 3.5 tons operating on 2,400 kilometers of selected highway corridors.
By the far the most ambitious scheme to date has been the one unveiled by the Dutch authorities, with an objective to charge all vehicles per kilometer driven over that nation’s entire road network by 2018. While plans for its deployment were halted last November due to a change of government, the scheme has been monitored with great interest by transport officials and political leaders in other countries.
Authorities in the various EU member states are examining the pros and cons of various technologies in order to ensure the profitability and viability of their respective approaches to road user charging. Satellite-based RUC schemes have a number of perceived advantages where application over larger geographical areas and a wider range of vehicle classes is being considered.
There is a general consensus that GNSS based road pricing main advantage is the flexibility: the scheme can be adapted over time, can cover quickly and easily additional roads, and can permit innovative, adaptive pricing strategies. On the other hand, there is a presumption that less fixed infrastructure, and therefore, a corresponding reduction in costs, is an objective.
However, although the technology to implement a GNSS pricing scheme exists, doubts about the technical and economic effectiveness of a large-scale application have persisted — especially with respect to the accuracy, integrity, availability, and continuity requirements critical to managing the balance between driver overcharging and loss of revenue to the road operator.
Objectives of GINA Project
This is the challenge to which the GINA (GNSS for INnovative Road Applications, www.gina-project.eu) project is trying to respond. Co-funded by the European Commission under the 7th Framework Program for Research and Development, managed by the European GNSS Agency (GSA) and coordinated by GMV, this two-year project started in March 2009 and involves 12 partners from 7 countries that cover the whole value chain.
GINA proposes to address the main aspects that can accelerate a large-scale adoption of road pricing and other value-added services (VAS) based on the use of EGNOS, and, in the future, Galileo.
The project aims to do this by, firstly, investigating the factors that could enable the application of GNSS-based road pricing and VAS on a national scale; secondly, by carrying out a nationwide demonstration of GNSS-based road pricing and VAS in the Netherlands to demonstrate and quantify the benefits of the European Geostationary Navigation Overlay Service (EGNOS) for nationwide road pricing schemes; and, thirdly, disseminating the project results, paving the way to commercial exploitation.
GINA revolves around the large-scale demonstration of RUC and VAS based on the use of GNSS using the definition of the Dutch system as a reference for the core project activity. In evaluating its performance, the GINA project uses the specifications that were defined for the Dutch Ministry of Transport’s ABvM (“Anders Betalen voor Mobiliteit” or “Alternative Payment Method for Mobility”) RUC model.
The project is working to demonstrate what the added value of the use of European GNSS (EGNOS and the Galileo system now under development) is for these applications in the road sector.
From a technical perspective, GINA capitalizes on the added value of EGNOS position integrity for road user charging and how this benefit applies to other road applications. A particular focus is on protecting users against the incorrect charging that can sometimes arise from large position errors and service interruptions resulting from the use of a single unaugmented GNSS system, such as the current GPS or GLONASS civil services, which lack a built-in integrity capability.
The trials were defined during the first part of the project and were carried out during 2010. The next section provides more details on objectives, equipment, infrastructure involved, use of reference systems, scenarios description, procedures for analyzing the data collected in the field trials, and some analysis of the results.
Trials Preparation and Definition
The UK-based Transport Research Laboratory (TRL) has practical experience and knowledge of the proposed Dutch road pricing scheme and developed a comprehensive testing program for the GINA project. The test program consisted of two major elements — exhaustive trials and end-to-end trials — that aimed to evaluate various aspects of the GINA RUC scheme.
The goal of both trials was to determine whether EGNOS (and, later, Galileo) can improve RUC systems in terms of charging reliability and accuracy. Further, the “exhaustive” trials were designed to test the performance of GINA on board units (OBUs — see inset photo above, right) based on GNSS/EGNOS technology in realistic and challenging circumstances.
The GINA OBU is based on a unit that provides a full set of hardware interfaces for communication, such as a UMTS/GPRS modem, coupled with a 50-channel, single-frequency GNSS receiver. The OBU has an embedded Linux OS based processor that runs navigation software providing integrity information via a SISNET server connection and interfaces to various sensors, such as a CANBUS, which provides odometer information that improves OBU performance in terms of distance measurement.
The OBU’s performance was measured against a positioning, attitude, and navigation reference systems that incorporated a dual-frequency GNSS receiver, an inertial measurement unit (FM-SAS), datalogger, control and display unit, and postprocessing software. The reference system used differential (DGPS) signals for better accuracy.
The GINA project also seeks to assess the potential for using EGNOS to improve charging accuracy and reduce — down to an acceptable limit — the risk of overcharging road users.
The “end-to-end” trials sought to facilitate a large-scale pilot of an RUC scheme and assess how participants perceived it. This second set of trials also aimed at testing the communications, OBUs, and back office operation over an extended period of time. The next sections take a closer look at each type of trial.
Exhaustive Trials
Exhaustive trials used two test vehicles, both of which were equipped with accurate reference inertial navigation equipment and a typical OBU as supplied by GMV. Vehicle I, the main GINA project–controlled test vehicle (see accompanying photo of “Furgo”) was driven along defined routes and schedules, according to a strict test plan.
Vehicle II was owned and controlled by NAVTEQ, a U.S.-based supplier of navigable map databases that is also a member of the GINA consortium. This vehicle was driven along routes in the Netherlands in May-June 2010 according to the road surveys that NAVTEQ personnel were performing at the time; GINA had only limited influence on the routes taken. Data from this vehicle were made available for analysis and comparison for the trials.
The reference systems provided “truth” data against which the GINA OBUs could be compared and were selected in a way that would provide distance and location measurement information of at least an order of magnitude more accurate than that of the OBUs under test.
The following description concentrates on the activities of Vehicle I, which provided the bulk of the data from the exhaustive trials.
Vehicle I testing was carried out during March 2010 and lasted for four weeks. TRL defined three separate test routes designed to be representative of the driving conditions and environments experienced by typical drivers in the Netherlands. The routes incorporated urban, motorway, and rural sections in various combinations.
The three routes were as follows:
- Route 1 – This route covered a hypothetical trip(s) from Amsterdam Schiphol airport to the center of Amsterdam and around the Amsterdam ring road. It included a set of challenging environments that could typically be expected in an urban area as well as tunnels and motorway stretches. The center of Amsterdam is a particularly challenging environment for GNSS-based systems due to a large number of tall buildings and narrow streets that create urban canyons.
- Route 2 – This route passed through the center of The Hague. It included sections through the highly built-up area of The Hague as well as general roads around the city.
- Route 3 – This route encompassed a long section of a road running parallel to a motorway and would test the OBUs ability to correctly identify the correct driven road. Further, it also followed roads through the highly industrial sections of the city that included many tall office buildings, urban canyons, bridges and tunnels.
On each route a number of geo-objects were defined — a total of 51 overall, consisting of realistic geo-objects that might be expected in a real charging scheme, for example, long road segments or large motorway cordons (see Figure 1). These were selected in order to facilitate analysis of geo-object recognition and charging performance.
Within this group, we also defined a sub-set of challenging geo-objects designed to test the limitations of GNSS technology in signal-challenged locations where satellite reception was anticipated to be poor.
Each route was designed to take approximately 1 hour and 20 minutes to complete. The trials involved at least 20 repetitions of each route in order to ensure that sufficient data would be available to make statistically significant conclusions regarding the potential benefits of EGNOS.
At the end of each day, data from the GINA OBU were automatically uploaded to an Internet-based location-based services (LBS) platform in order to minimize the chance of data loss.
Once the trial campaign was over, the collected data from the GINA OBU were postprocessed in order to prepare the data for analysis. The postprocess procedure can be briefly described as follows:
The GINA OBU records relevant GNSS data in binary format (due to storage space requirements). These data contain all the required information for GINA analysis including position, speed, distance traveled, and so forth.
Once the data enter the postprocess phase, two different files are generated for each of the configurations to be tested (up to four in the framework of GINA). The first of these files includes the following data:
- Timestamp (gps week, gps tow)
- position information (latitude/longitude) without integrity information
- distance travelled (meters)
- geo-objects detection flag. (Boolean result True/False)
The second file includes the same set of information with the added value of integrity for the positions included in the first file, in the form of 0.1, 1e-2 and 1e-4 thresholds, meaning:
- 0.1 — integrity valid 99 out of 100 times, or 99 percent
- 1e-2 – integrity valid 99.9 out of 100 times, or 99.9 percent
- 1e-4 – integrity valid 99.99 out of 100 times, or 99.99 percent.
For all the geo-object detection streams present in the first file, the same information set is checked in the second file in order to ensure that the different epochs in the information set have the required integrity value. With this final information, the OBU output data file is formatted.
At the same time the information from the position, attitude, and navigation unit is stored in a different file, which includes positions and distance information recorded by the unit. This information is considered as a reference without any integrity computation due to the precision provided by the reference unit itself.
By the end of this postprocess phase, up to five files have been created: one for the SPAN unit and four for the OBU, one for each configuration to be tested.
In order to allow direct comparison of EGNOS- and non-EGNOS–enabled devices, during the project we chose an approach in which a single GINA OBU was installed in trial Vehicle I that was capable of producing simultaneously four “real-time” streams of position estimate data:
1. using the GPS-only signal (G)
2. using GPS signals and odometry data from the vehicle’s controller area network bus (CANBUS) data combined (C)
3. using GPS and EGNOS signals combined (E)
4. using GPS, EGNOS, and CANBUS combined. (A)
Each of these data sets for all routes was then compared against the truth data from the reference system.
Vehicle II, which NAVTEQ personnel operated during the trials, collected data on undefined routes. Because it was not possible to use Vehicle II to travel repeatable routes with a high number of repetitions, data collected from this part of the exhaustive trial was handled differently from that obtained from Vehicle I.
A separate set of geo-objects was defined for Vehicle II that aimed to maximize the likelihood of the vehicle entering a geo-object. Two different types of geo-objects were defined: large areas encompassing municipalities where Vehicle II was expected to be operating and smaller road segment geo-objects inside those municipalities.
Because a connection could not be established to the CANBUS in Vehicle II, two streams of data were generated:
1. using only the GPS signal
2. using GPS and EGNOS signals combined.
We collected Vehicle II data during June 2010. Following completion of the data-gathering phase, data from the OBU was postprocessed and made available for analysis and evaluation.
End-to-End Trials
These trials involved equipping 100 participating vehicles with GINA OBUs. ARVAL Netherlands, a member of the GINA consortium and the subsidiary of the second largest car-leasing company by size in Europe, provided the vehicles. Volunteer drivers were selected from ARVAL’s client portfolio and from ARVAL employees, who showed interest in joining the trial.
Data were gathered from those vehicles over a six-month period that began July 2010. The purpose of these trials was to assess the overall functionality of the GINA system in terms of reliability, communications, and billing system, as well as to gather user perceptions. A selection of value-added services was also tested with user feedback gathered from these experiences.
The participating vehicles did not follow any pre-defined routes, instead, they were used for regular commuting and business travel. TRL defined a separate set of geo-objects in locations that would maximize the number of times participating vehicles might enter one or more of them, in the absence of defined routes, during the six-month trial. These geo-objects consisted of large cordons matching municipalities’ boundaries in the Netherlands (see Figure 2) and smaller road segments located near participants’ places of work.
Due to privacy laws, no second-by-second data was collected about the participants’ trajectories. Instead, summary reports were produced detailing the total distance traveled and the geo-objects entered. Based on those data regular bills were produced and made available to the participants via an online user interface.
Participants’ feedback was gathered regarding the ease of use of the scheme, the billing, and the tariffs proposed. However, participants in the trials made no actual payments; the billing system was implemented as a way to inform the participants about their use of chargeable road infrastructure.
Following the completion of the trial campaign a full set of results will be published.
Preliminary Analysis of Exhaustive Trials
The analysis carried out thus far on data from the exhaustive trials collected from Vehicle I shows that the types of supporting technologies used in conjunction with GPS — that is, the CANBUS odometer and geo-fencing based on position integrity — had a significant effect on the accuracy of position and distance measurement.
Geo-fencing involves the establishment of a virtual perimeter for a geographic area. In the case of the GINA design, this includes horizontal protection levels (HPLs) that provide a margin of the error associated to the calculated GNSS positions to ensure that an RUC fee is correctly charged. A vehicle is charged only when one or more HPLs — in effect, error boundaries — is totally inside the geo-object.
In terms of distance measurement accuracy, those configurations of the OBU that included a CANBUS input performed the best, achieving a relative distance deviation (RDD) over all routes of 0.23 percent (for the 95th percentile). The RDD (for 50th percentile) was 0.05 percent for CANBUS-connected configurations and between 0.31 percent and 0.33 percent for configurations without a CANBUS connection. (See Figure 3)
The addition of EGNOS, in this case, had little effect on the distance measurement accuracy, and the CANBUS odometer was the dominating factor in providing accurate distance measurement.
Concerning absolute positioning accuracy, results for 95th percentile are considered for comparison (see Figure 4). Comparing configurations C (GPS + CANBUS) and G (GPS data only) shows that the results appear to be identical, with the CANBUS/odometer having no effect on the accuracy of determining position. This was expected, as the CANBUS/odometer output — being a dead reckoning measurement — should only have an effect on distance measurement and not position accuracy.
Configuration E (GPS + EGNOS), represented by the black line in Figure 4, performed worse than the other three configurations. This configuration applied minimum operational performance standards (MOPS) techniques to the EGNOS data for position determination while Configuration A (GPS+EGNOS+CANBUS odometer), also using EGNOS, employed Kalman filter techniques.
These MOPS were developed by the civil aviation community to be used with satellite-based augmentation systems such as the U.S. Wide Area Augmentation Systems (WAAS) and EGNOS.
Because we have already shown that the CANBUS odometer has no effect on the positioning accuracy, this suggests that the use of EGNOS MOPS may not be suitable for land vehicles operating in urban environments, which that present in a majority of the routes. For these specific uses, a customization of EGNOS MOPS to cope with challenging urban environment should be required.
The quality of EGNOS data should be treated separately from the adequacy of MOPS standards (which prescribe how such data must be handled) for urban environments. By comparing configurations C and A (both Kalman filtered, and hence not compliant with MOPS standards) one sees that Configuration A (where EGNOS data was Kalman filtered) produced the most accurate positioning at the 99th percentile and also had the lowest maximum position error of 109 meters.
As for the performances of the GINA system concerned with the identification of geo-objects and the measurement of the distance inside these geo-objects, we found that, for official geo-object types (such as long road segments), all of the OBU configurations detected all of the geo-objects successfully. This means that at least one position was determined inside the perimeter of each official geo-object.
Additionally, none of the geo-objects of any type (official or challenging) was wrongly detected. This can be attributed to the use of GINA’s geo-fencing technique, based on position integrity.
This performance is crucially important for any charging scheme, both in discrete and in continuous schemes, where the correct identification of geo-objects might have a beneficial effect in reducing the charging error associated with the RUC system.
Geo-Object Definition and Road User Charges
Our analysis also sheds some light on the most appropriate way to define geo-objects in an RUC scheme. The way in which geo-objects were defined played an important role in how likely they were to be successfully detected by an OBU.
Narrow road segments (corridors) and short geo-objects (less than half a kilometer in length — the so-called challenging geo-objects) indeed proved to be very challenging for all OBU configurations: more than 50 percent of distance travelled within those geo-objects was regularly missed by all OBU configurations. However, for geo-objects defined as realistic and representative of geo-objects in a practical RUC scheme (what we have referred to as official geo-objects), all OBU configurations performed well in terms of correctly identifying geo-objects and measuring distances.
As mentioned previously, all of the official geo-objects were detected by all GINA OBU configurations, and no geo-objects were wrongly detected, with total missed distance in all official geo-objects being only 1.64 percent. Distance incorrectly identified as traveled inside official geo-objects corresponded to less than 0.1 percent of the total geo-object distance.
Overall, performance in distance measurement and official geo-object identification for the GINA OBU resulted in the overall charging deviation being between -0.65 and -0.74 percent over all journeys, resulting in zero overcharging over the corresponding dataset (Figure 5).
Two different methods were considered for determining the distance driven inside geo-objects. The first requires that a minimum of two positions be registered inside the geo-object boundaries in order to be able to calculate the distance between the consecutive positions inside that geo-object.
Therefore, with this method the sum of distances between adjacent positional fixes provides the total distance within the geo-object. This method produced the results that we have just been presented concerning charging performances.
This primary method is considered very appropriate to use in a real, driven-distance charging scheme. However, recognizing potential associated drawbacks, we also applied an alternative method, which led to slightly different results.
The alternative method uses the first position recorded on entering a geo-object and the first position recorded upon leaving the same geo-object as, respectively, the start and end points for calculating the measured distance inside the geo-object. In other words, this calculation is the sum of all increments of distance between adjacent points between this start and end point.
With this second method, the overall charging deviation ranged between -0.57 and -0.44 percent over all journeys, with zero overcharges being assessed for the corresponding dataset.
Table 1 compares the results from the alternative method with results from the primary method. Taking the entire data set into consideration, the main method clearly produced more undercharging than the alternative method, as seen in Table 1.
In any case, the charging results always met the specifications defined in the ABvM system.
EGNOS MOPS Performance in Inter-Urban Areas
Given the performances obtained in combined environments (especially those challenging scenarios such as the urban ones) and in order to better understand how EGNOS-enabled configurations perform in non-urban environments, we selected and analyzed a subset of the data gathered from Vehicle 1 (“Furgo”) during the exhaustive trials.
This subset consisted of a single motorway section from Route 1, which we analyzed independently from the rest of the data from that route. All repetitions of Furgo’s trips along this section of the route were considered and produced the following results.
As shown in Figure 6, OBU configuration E (GPS + EGNOS using MOPS) appears to have produced smaller errors in position accuracy than other configurations on the motorway section. Configuration E, at the 95th percentile, produced errors of up to three meters, compared with errors of up to six meters for other configurations.
This result appears to be in line with previous results obtained, which suggested that urban environment may have had a negative impact on the accuracy of position determination for Configuration E, which uses the aviation-oriented MOPS.
We also evaluated the results using the metric of horizontal protection levels.
At the 50th percentile, HPLs from all OBU configurations were up to 7 meters in radius. For configuration E (EGNOS using MOPS), HPLs were smaller than 15 meters at the 99th percentile and had an associated integrity risk of 3×10-4. (See Figure 7.)
These results seem to suggest that EGNOS (using MOPS) is able to provide smaller HPLs and significantly lower integrity risk in inter-urban conditions than in urban conditions.
The mean charging deviation appears to be between -0.07 and -0.15 percent over all journeys. The charging deviation at the 95th percentile is between 0.21 and 0.38 percent, as can be seen in Figure 8, where the probability density function of having an error of a given size is modeled.
Again, the addition of CANBUS seems to be the overriding factor in ensuring that road user charges for a given distance are as accurate as possible.
Conclusions
The GINA project is being carried out at a time when European policy makers are debating the possibility of employing road user charging in a more innovative and holistic way, so that the flexibility of GNSS-based schemes could prove a key factor of success.
The project tackles the adoption of EGNOS (and Galileo in the future) in the road sector, from technical, economic, and other perspectives. The project addresses this challenge by implementing a large-scale nationwide demonstrator for road charging and value-added services, using the requirements of real end users (the Dutch government and a car leasing company, ARVAL). This is complemented by a thorough context analysis (with particular emphasis on business aspects) and an intensive dissemination strategy.
The project revolves around the concept of position integrity, which is used as a road user protection mechanism to meet the performance expected for a distance-based RUC scheme.
The demonstrator set up in the project was split into two levels (exhaustive analysis and end-to-end analysis), which allowed the recording and analysis of data. From this we have reached some useful conclusions with respect to the use of European GNSS in the road sector, particularly as regards the added value of position integrity for RUC and VAS.
The analyses carried out confirmed that GNSS is a reliable tool for different RUC schemes and that GINA proposed technology (geo-fencing based on position integrity) allows distance-based charging with good performances and a simple affordable solution. Therefore, European GNSS (e.g., EGNOS) and other GNSS-centered techniques can be used to improve performances and reliability.
Analysis of data gathered during exhaustive trials using a GMV-operated vehicle showed that the addition of a CANBUS/odometer connection to a GINA OBU can significantly increase the overall accuracy of distance measurement.
In terms of GINA’s performance in identifying geo-objects, the analysis showed that for official-type geo-objects, (those that could be expected to be defined in a realistic charging scheme), the GINA OBU (in all configurations) was able to detect all geo-objects and did not incorrectly identify any geo-objects, official or challenging.
The performance of the GINA OBU resulted in a minimal charging deviation, (considering only the official-type geo-objects) with no instances of overcharging.
We used a subset of the data to better understand the performance of the system in combined environments (and especially those containing challenging scenarios, such as the urban areas) and in order to understand how EGNOS-enabled configurations perform in non-urban environments.
The results obtained through this additional analysis showed that an OBU configuration of GPS + EGNOS (and using MOPS criteria for calculating positions) appears to have produced smaller errors than other configurations on the motorway section. The same conclusion can be obtained when analysing size of HPL and integrity risk, as it seems that EGNOS (using MOPS) is able to provide smaller HPLs and significantly lower integrity risk in inter-urban conditions than in urban conditions.
The charging deviation in motorways sections shows very satisfactory results, totally aligned with the specifications defined by ABvM. In challenging urban environments, the use of EGNOS MOPS doesn’t appear suitable. EGNOS MOPS should be customized to cope with these challenging environments where local errors prevail.
The analysis also showed that using small geo-objects, especially those defined as narrow corridors, can significantly increase distances missed by an OBU. Experience from the exhaustive trials suggests that the geo-objects should be as large as possible and as wide as adjacent roads would allow. Where possible, geo-objects should be defined as cordons as opposed to corridors.
As a general conclusion, GINA has proposed a GNSS-based technology that is suitable for:
- different kinds of charging schemes: both discrete and continuous (distance-based) RUCs
- road and urban environments
- implementation without need for on-board cartography
- using a simple and inexpensive on-board unit
- offering guaranteed performance
- implementation in different OBU architectures, either “thin,” “fat,” or “smart”
- satisfying the performance framework defined by a system as demanding as the Dutch ABvM
The GINA project has tried to address the strengths of European GNSS and to identify weaknesses that should be addressed as a next step. These conclusions will shed some light on still open points associated with the use of GNSS for RUC schemes while paving the way to the adoption of Galileo in the road sector.